The Product Management Blog
By sharing your email, you agree to our Privacy Policy and Terms of Service
Featured
Discover our posts
Showing 9 of 597
Analytics
12 Customer Engagement Metrics for Revenue Retention
Learn how to track 12 key customer engagement metrics for insights that boost retention, reduce churn, and protect your bottom line.
Career Development
Product Analyst Career Guide: Skills, Salary, Steps
Learn what a Product Analyst does, key skills, salary insights, and how to become one. Compare roles like Product Manager and Data Analyst.
Leadership
Agile Organization: Key Traits of Top Performers
Discover what makes an agile organization thrive. Learn key traits, team structures, and tips to build a truly agile organization.
User Experience
Emerging UX Trends Every Product Leader Should Watch
Explore key UX trends for 2025. Learn how AI-driven research and continuous discovery help product leaders build smarter, user-focused products.
Product Strategy
Product Evangelist: These Are the Champions
Learn what a Product Evangelist is, why the role matters, who should own it, and how to master product evangelism in your team.
Product Fundamentals
Product Risk: What It Is and How to Manage It
A clear guide to product risk: how it works, why it matters, and what product teams can do to keep it in check.
Product Fundamentals
Product Viability: Find Out If Your Idea Has Legs
Dig deep into what product viability means, why it matters, who's responsible, and how to assess if your product idea is worth building.
Leadership
Growth Team Structure: How to Build High-Value Teams
Find out what a growth team is, how it works, and how to build one — from key roles and structure to real examples from top companies.
Analytics
Product Success: 13 Metrics That Measure What Matters
Learn how to evaluate product success, which metrics to track, and how to build a strategy that blows past the competition.
Subscribe to our newsletter
A newsletter that will make you a better Product Manager
By sharing your email, you agree to our Privacy Policy and Terms of Service
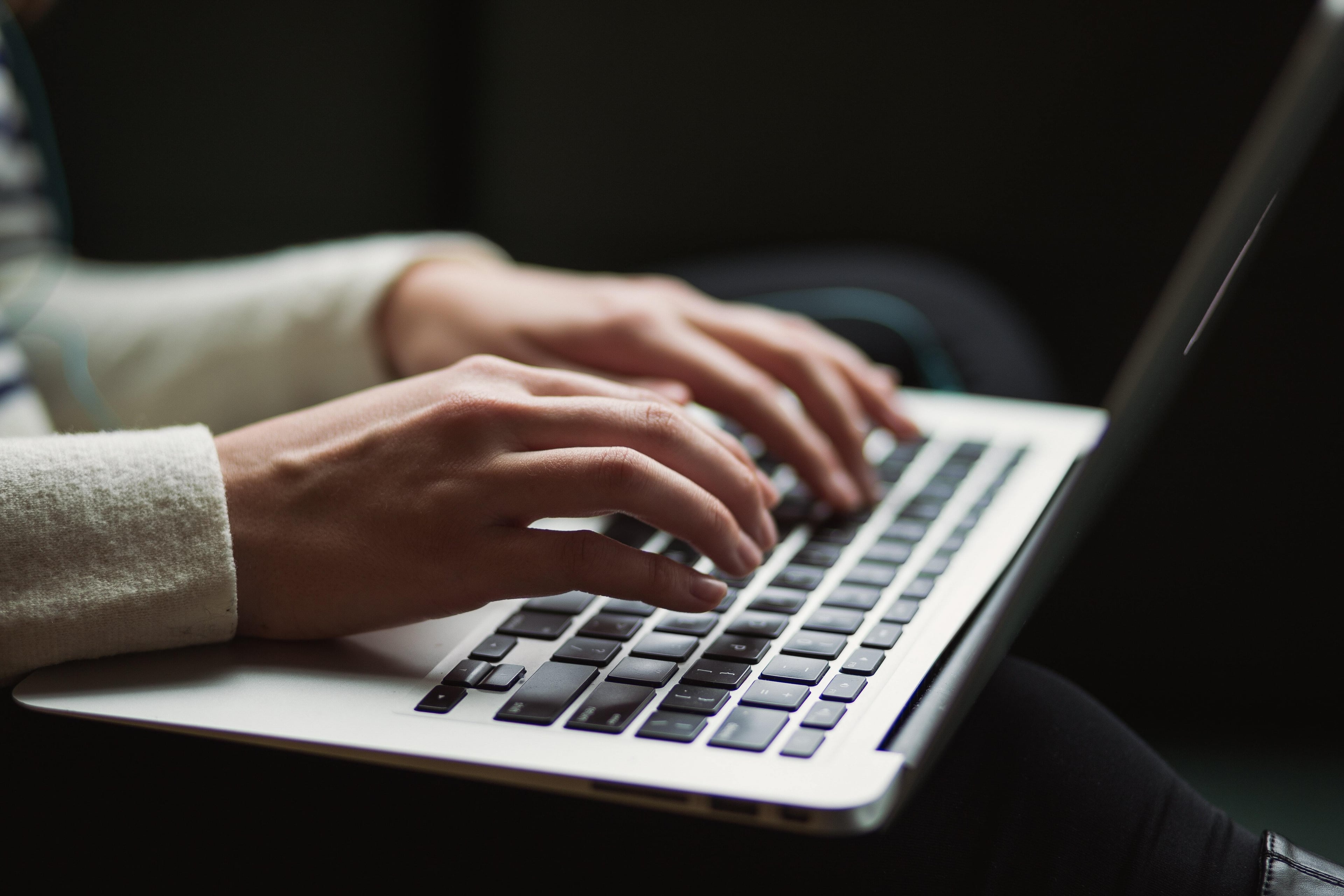