Updated: May 6, 2024- 8 min read
With Mckinsey forecasting AI and machine learning to create $1.7 trillion dollars of business value in the retail industry (roughly 12% of all sales), eCommerce businesses must prioritize this technology if they don’t want to be left behind in this sink-or-swim sector. In this article, you’ll learn how to leverage the power of machine learning in eCommerce with five practical use cases and real-world examples from industry leaders.
Plus, we’ll be sharing actionable tips to unlock the power of machine learning in your organization.
But first, let’s do a brief recap:
What is machine learning?
Machine learning is a cutting-edge subset of artificial intelligence that empowers computer systems to analyze vast amounts of data, identify patterns, and make intelligent decisions without explicit programming.
At its core, machine learning is driven by algorithms designed to optimize models through experience and continuous learning. These algorithms allow computers to recognize speech, image patterns, recommend products, detect fraud, and perform many other tasks more efficiently than traditional, rule-based systems.
The power of machine learning lies in its ability to constantly adapt and improve, offering the promise of revolutionizing industries from healthcare to finance, and from transportation to eCommerce.
Custom team training
Learn how our training sessions can give your organization a competitive edge.
Schedule a call5 use cases of machine learning in eCommerce with real-world examples
1. Segmenting users for targeted marketing
User segmentation involves categorizing customers based on common attributes or behaviors. Machine learning algorithms can analyze vast user data sets to create meaningful segments, enabling businesses to deliver personalized content and offers to each group.
Real-world example: ASOS, one of the UK’s largest online fashion retailers, uses machine learning to segment users by creating clusters of products based on customer preferences, identifying high-value customers, predicting intention to purchase and value of the purchase, predicting the likelihood of a customer churning, and understanding customer value.
By leveraging advanced analytics, artificial intelligence (AI), and machine learning (ML), ASOS has been able to adapt to changing consumer behaviors and preferences and redefine the customer experience, driving engagement and growth in the process.
2. Personalized product recommendations
Machine learning-powered recommendation engines are a cornerstone of eCommerce personalization. By analyzing a user's historical behavior and preferences, these engines suggest products that are highly likely to interest the customer and increase engagement. What’s more, McKinsey’s "The Future of Personalization" paper found companies implementing ML-based product recommendation systems increased revenue by 5–15%.
Real-world example: Walmart employs advanced machine learning algorithms and data analysis to offer personalized product recommendations to its customers. When a shopper visits the Walmart website or app, the platform collects data on their browsing history, purchase behavior, and even location.
Using this information, Walmart's recommendation engine suggests products that align with the shopper's interests and needs. For example, if a customer has been searching for baby products, Walmart will show them relevant items such as diapers, baby clothing, and toys.
By leveraging machine learning, the retailer's product recommendations engine can now deliver more relevant suggestions to shoppers by drawing from both Walmart's online and in-store transactional data –not only making the online shopping journey more convenient but also increasing the chances of cross-selling and upselling.
What’s more, since Walmart started using deep learning AI to make smarter substitutions in online grocery orders, it’s seen shopper acceptance of substitutions increase to more than 95%.
In summary, Walmart's implementation of personalized product recommendations with machine learning has helped the retailer: remain competitive in the fast-changing retail environment, drive engagement, encourage repeat visits, and ultimately increase sales.
3. Optimizing inventory management and stocking decisions with predictive analytics
Machine learning can forecast demand for products, allowing businesses to optimize inventory levels. By ensuring products are in stock when customers want them, eCommerce platforms can enhance user satisfaction and engagement.
Real-world example: Amazon employs machine learning to optimize inventory management and stocking decisions on a colossal scale. The e-commerce giant uses predictive analytics to forecast demand for an extensive array of products. Amazon factors in various variables, such as historical sales data, customer browsing and purchasing behavior, seasonality, and even external factors like weather events that may affect demand.
This enables Amazon to strategically position products across its vast network of fulfillment centers, ensuring that popular items are readily available for quick delivery. Leveraging machine learning in inventory management enhances the customer experience by reducing shipping times and ensuring product availability, thereby increasing engagement and fostering customer loyalty.
4. Implementing ML-powered chatbots
When done right, chatbots can be a boon for eCommerce companies. Machine learning-powered chatbots can provide instant responses to customer inquiries, offer round-the-clock support, reduce the workload on customer service representatives, as well as collect customer data and insights that can be used to improve the customer experience even further.
Real-world example: H&M’s AI chatbot on Kik takes the role of a personal digital stylist and helps customers save time by guiding them through the online store areas that align with their purchase desires.
To do this, the H&M chatbot asks users a series of questions to understand their tastes and preferences, and to make the process more engaging, it also sends pictures of clothes to help users answer style questions.
The chatbot identifies the user's gender and style, suggests an outfit that matches their style, and provides the total price of the outfit. If the user dislikes the suggested outfit, the chatbot gives them different options, allows them to save the outfit, and gives them the opportunity to socially share the outfit. Not only that, the chatbot remembers each user's tastes and preferences and uses this for retargeting customers in the future with better recommendations.
From enhancing the customer experience to driving engagement, H&M’s chatbot has it covered.
5. Developing visual search features
Machine learning can enable visual search capabilities, allowing users to search for products using images rather than text. This feature simplifies the shopping experience and increases engagement.
Real-world example: Pinterest utilizes visual search technology to enable users to discover products similar to those they find in images on the platform. This feature encourages users to explore and engage with products.
By using visual search, Pinterest helps users discover new ideas and products, which increases engagement. Additionally, visual search helps Pinterest stand out from other social media platforms and provides a distinct business model and strategy.
Learn More
Discover groundbreaking retail innovation strategies that are reshaping global consumer experiences. Don't get left behind – learn, adapt, and lead your market.
Read now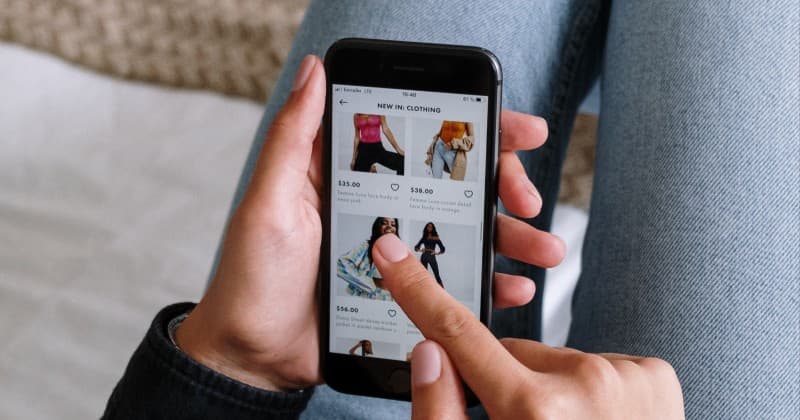
How to leverage machine learning in eCommerce
As you’ve seen, machine learning can improve customer experience, boost engagement, drive sales, and remain competitive in the fast-changing eCommerce sector. Now let’s explore some practical steps you can take to unlock its potential in your organization:
1. Gain a deep understanding: Before diving into the world of machine learning, it's crucial to have a comprehensive understanding of the technology. Start by researching the current state of machine learning, its capabilities, and how it works. This foundational knowledge will help you make informed decisions when implementing machine learning solutions.
2. Study the competition: Study how other eCommerce businesses are using AI and machine learning to their advantage. Explore machine learning solutions in the market and analyze their impact on various aspects of eCommerce, such as personalization, recommendation engines, and customer support. Learning from successful use cases can provide valuable insights for your own strategy.
3. Acquire expertise: It's common for eCommerce businesses to lack in-house expertise in machine learning. Consider bringing in outside experts, whether through hiring new talent or consulting with professionals who specialize in AI. These experts can guide you in implementing machine learning solutions effectively and efficiently.
4. Identify specific problems: Machine learning should be applied to solve specific, well-defined problems in your eCommerce operations. Rather than aiming for broad objectives like "increasing sales," pinpoint precise challenges. For instance, focus on improving retention rates among first-time buyers or optimizing product recommendations. Clear problem definitions lead to more targeted and effective solutions.
5. Assess technology gap: Understand your organization's technology and capability gap. Consider your existing tech stack, infrastructure, and the resources available for implementing machine learning. Avoid setting overly ambitious goals that may exceed your current staffing or technical capabilities.
6. Build a dedicated team: Building and managing machine learning solutions requires a dedicated team. Assemble a group of professionals with the necessary skills and knowledge to execute your machine-learning vision. This team should include data scientists, engineers, product managers, and domain experts who can collaborate effectively to achieve your goals.
7. Start Small, Scale Wisely: Begin your machine learning journey with manageable projects and gradually scale as you gather data and insights. Starting small allows you to learn from initial implementations and make necessary adjustments. As you gain confidence and see positive results, you can explore additional applications of machine learning in your eCommerce business.
Updated: May 6, 2024