Updated: April 25, 2024 - 12 min read
The rise of AI-powered organizations marks a significant shift in how products are conceived, developed, and managed, as well as the skills and approach you need as a Product Manager (PM). But fear not. We’ve put together this comprehensive guide to help you deepen your knowledge of AI in Product Management and develop the skills you need to become a world-class AI PM.
In this guide we’ll cover:
The key stages of product development in AI-powered organizations
The unique challenges of AI Product Management
The skills you need to become a kick-ass AI Product Manager
Tips and resources to develop a career in AI Product Management
Editorial note: This post is based on the webinar PM in AI-First Organizations by eBay AI Product Leader, Yogesh Soni, and contains additional insights and examples from the Product School team. You can watch the webinar in full below.
Before we dive into the various stages of product development in AI-powered organizations, here’s a quick reminder of the importance of AI and its impact on Product Management.
Why should PMs care about AI-driven Product Management?
From enhancing customer experiences to optimizing operational efficiencies, AI's potential is vast. And it's not just confined to a niche sector. AI is everywhere. Artificial Intelligence is playing a pivotal role in sectors as diverse as entertainment, healthcare, finance, and more – making huge waves in the global economy.
According to McKinsey, AI and AI-based applications are expected to unlock tremendous economic potential – about 13 trillion dollars by 2030 – boosting global GDP by around 1.2% per annum. To put this into perspective, the steam engine, a transformative technology in human history, added about 0.3% to GDP between 1850 and 1910.
Gaining expertise in AI-driven Product Management isn't just about staying relevant; it’s about being at the forefront of a technological revolution that is reshaping industries, creating new market opportunities, and redefining how businesses operate.
Become An Expert In AI-Driven Product Management
Unleash the power of AI in your Product career! Learn how to make data-driven decisions, accelerate product development, and craft products that resonate with market needs.
Read Now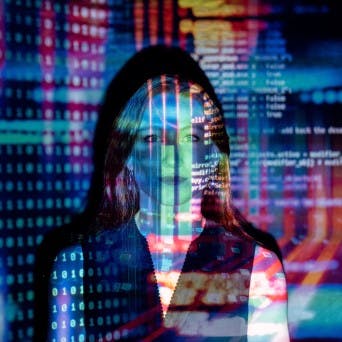
Key stages of product development in AI-powered organizations
When it comes to AI product development, the product lifecycle undergoes a fundamental shift. Unlike traditional software development, AI-based systems require a different approach, emphasizing data, continuous learning, and adaptability.
Let’s take a closer look using the example of an AI-powered spam detection service.
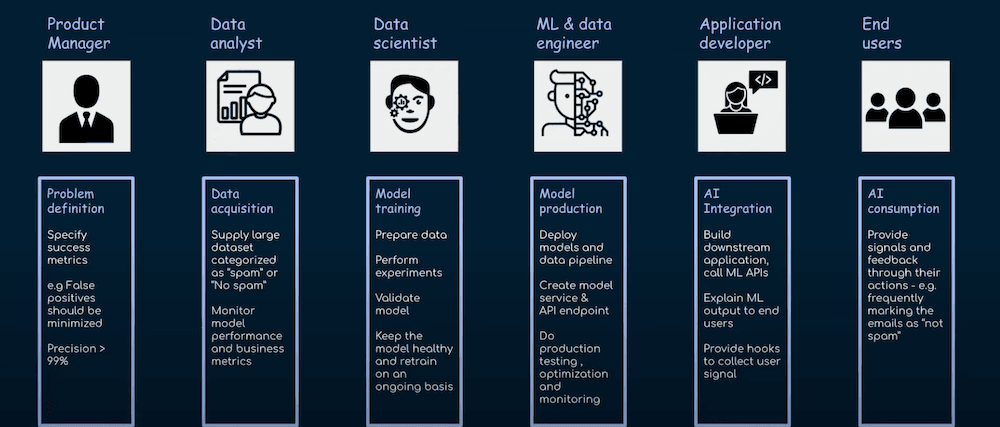
1. Problem definition
At the start, a business stakeholder (often a Product Manager) defines the business problem that the AI solution aims to solve. In our spam filter example, the problem and need are clear: we receive numerous emails, many of which are spam, and we need a service that filters these effectively. This service would check each incoming email, categorize it as spam or not, and route it to the appropriate folder.
Important: In AI, apart from defining the problem statement, it's essential to define the success metrics clearly. For instance, in spam detection, minimizing false positives is critical. You wouldn't want an important email, like a job interview notification, to be incorrectly marked as spam.
2. Data acquisition
Once the problem and metrics are defined, data analysts acquire the necessary data to train your AI model. For a spam filter, this involves collecting and labeling a large number of emails as spam or not. This data can come from various sources, including existing services like Gmail, which have access to vast quantities of emails.
3. Model training
Data scientists then explore this data to identify the characteristics of spam emails, such as frequent mentions of 'free money'. They prepare the dataset, removing irrelevant information, and then train the model using this curated data. This process involves a lot of experimentation with different AI architectures. Once a model performs well on the validation dataset, it's considered ready for production.
4. Model production
In production, a machine learning engineer or data engineer ensures the model is integrated into a service, like an email system, and can handle the scale of operation, such as classifying millions of emails accurately and swiftly.
Models in production require continuous monitoring to ensure they don't degrade over time, a phenomenon known as 'drift'. If the model's effectiveness decreases, it needs retraining or updating. In some cases, models are trained continuously to adapt to changing patterns, like the evolving nature of spam.
5. AI integration
Application developers play a crucial role in integrating the AI model into the larger system, like an email service, and often need to provide explanations for the model's decisions. For example, Redfin, a real estate website in the United States, not only estimates house selling prices but also provides explanations for its pricing, increasing user trust.
6. AI consumption
Finally, end users are vital in the product development lifecycle. Their feedback, like flagging an email wrongly classified as spam, provides valuable data for improving the model.
Before Adding AI Features: What Every Product Manager Must Know
Discover the must-know considerations before integrating AI features to ensure a seamless and value-driven experience.
Read Now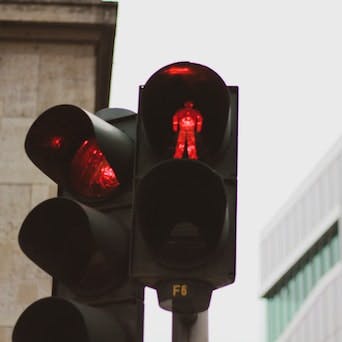
The unique challenges of AI Product Management
Now that we’ve got a high-level overview of the product development process in AI-powered organizations, let’s take a look at some of challenges AI Product Managers face.
Data sensitivity and quality
The adage "garbage in, garbage out" is particularly apt when it comes to training AI models. The quality and relevance of the data used in training directly impact the effectiveness and reliability of the AI system.
Sourcing high-quality data, ensuring its relevance, and maintaining its integrity are vital steps in developing AI solutions that are not only effective but also trustworthy. This involves rigorous data collection protocols, continuous validation, and verification processes to ensure that the data accurately represents the problem space and the environment in which the AI will operate.
Ethical considerations
Another critical challenge in AI Product Management is addressing the ethical considerations that arise with AI development and deployment. As AI systems often learn from historical data, they can inadvertently perpetuate existing biases present in that data.
It's the responsibility of AI Product Managers to recognize and address these biases, ensuring fairness and equality in AI decisions. This involves implementing strategies like using diverse data sets that encompass a broad spectrum of scenarios and demographics and deploying fairness algorithms that actively seek and mitigate biases.
Moreover, transparency in AI decision-making processes is crucial to build trust among users and stakeholders. AI Product Managers must work tirelessly to ensure that their AI systems are fair, transparent, and accountable, which includes being open about their capabilities and limitations, and providing recourse in case of errors or disputes.
Complex stakeholder management
The final significant challenge in AI Product Management is managing the complex web of stakeholder relationships. AI projects typically involve a variety of stakeholders, including data scientists, engineers, business leaders, end-users, and sometimes even regulatory bodies. Each group has its own set of expectations, objectives, and perspectives. Balancing these diverse needs and viewpoints requires a high level of diplomacy and strategic thinking.
AI Product Managers must navigate these relationships, ensuring clear communication and alignment of goals across all levels. This involves understanding the technical aspects well enough to communicate effectively with engineers and data scientists and translating these details into strategic business value for leadership and tangible benefits for end-users.
It's about building bridges between the technical and non-technical worlds, fostering a collaborative environment where all voices are heard and considered. In doing so, AI Product Managers not only enhance the development process but also ensure the resulting AI product aligns well with both business objectives and user needs.
The AI Product Management skills you need to develop
Taking these unique challenges of AI Product Management into account, it becomes evident that you require a special skillset to achieve success in this field. Let’s take a look at some of them:
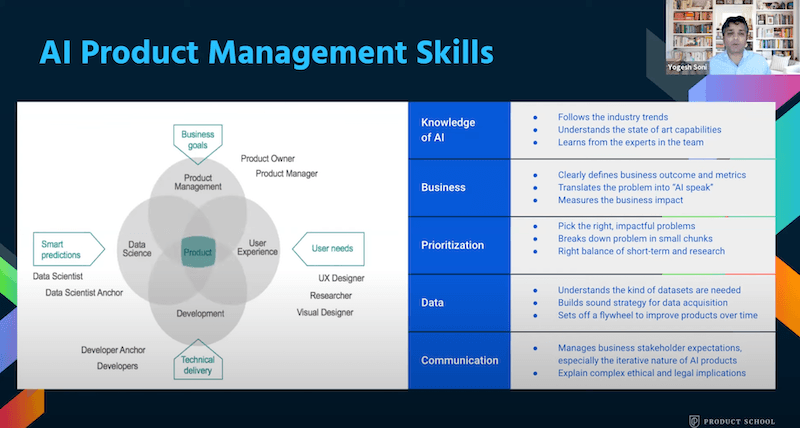
1. Knowledge of AI
No surprises here, but as an AI Product Manager, a profound understanding of AI and its capabilities is fundamental. This knowledge isn’t limited to the basic concepts; it extends to understanding the nuances of AI models, their development, and deployment. You don’t have to be an expert, but the ability to grasp the technicalities of AI helps in making informed decisions and effectively guiding the product development process.
Familiarity with the latest AI trends and technologies, as well as a strong grasp of how AI can be applied to solve real-world problems, is also crucial in this role.
2. Strategic Vision
Having a strategic vision is key to being a successful AI Product Manager. It involves looking at the bigger picture and understanding how AI can be leveraged to achieve the long-term goals of your organization. This means not only aligning AI projects with the company's mission and objectives but also foreseeing how AI can shape or transform future business landscapes.
A strategic vision helps in navigating the complexities of AI technology and ensuring that the products developed are not only innovative but also aligned with market needs and trends.
3. Prioritization
With numerous potential applications of AI, it's crucial to identify which projects or aspects will bring the most value to the organization. AI can be hyped, and senior stakeholders might have unrealistic expectations.
Defining the right problems, breaking them into manageable parts, and focusing on quick wins can create meaningful impact and garner more resources for future projects. This involves evaluating the feasibility, impact, and alignment of AI initiatives with business goals. Prioritization also means being agile and adaptable, capable of adjusting strategies as new information and AI advancements emerge.
How To Master The Art Of Prioritization In Product Management
Learn how prioritization shapes efficiency, productivity, and strategic focus. Plus, explore practical steps to balance immediate tasks with future goals.
Read Now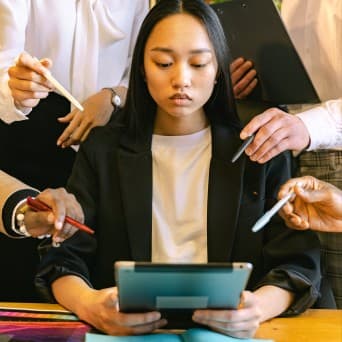
4. Data management
Since AI systems are only as good as the data they're trained on, understanding data acquisition, processing, and labeling is vital. You need to ensure that the data used is of high quality, relevant, and ethically sourced.
This makes proficiency in data management is essential for an AI Product Manager. Mastering this skill also includes being able to work with data scientists and analysts to refine data strategies, ensuring the AI models developed are accurate, efficient, and effective.
5. Communication
While effective communication is a cornerstone skill for all Product Managers, it’s paramount in AI-powered organizations. It's up to you to bridge the gap between technical and non-technical stakeholders, translating complex AI concepts into understandable language. You need to be able to convey the value and capabilities of AI systems to business leaders, while also understanding and addressing the concerns of engineers and data scientists.
Good communication fosters collaboration, aligns stakeholders, and ensures that the development process is smooth and the final product meets the expectations of all parties involved. What’s more, communication skills are not only crucial internally with stakeholders but also in explaining complex ethical and legal issues related to AI.
How to build a career in AI Product Management
Despite the challenges of a career in AI Product Management, it’s an exciting and rewarding field to work in. If you’re interested in transitioning into an AI Product Manager, here's how you can prepare:
Learn, learn, learn
The first step in your journey is to build a solid foundation of AI knowledge. And the good news is that Product School offers many FREE resources to help you on your AI learning journey. Get started here:
Check out these blog posts all about AI
Watch our AI video playlist. Containing over 70 webinars, panel discussions and presentations, this is a treasure trove of AI knowledge
Experiment with these top AI tools for Product Managers
Get hands-on experience
There's no substitute for practical experience. Engaging in AI projects or simulations gives you a tangible sense of how AI works in real-world scenarios. This could involve participating in guided learning labs, where you can build AI models yourself. Even simple projects, like creating a spam filter, can teach you a lot about the AI product development lifecycle.
If you're already in a tech role, seek opportunities within your organization to work on AI projects, or consider contributing to open-source AI projects to gain hands-on experience.
And of course, it goes without saying that seeking employment at an organization where AI is a central component of the product will give you the most opportunity to advance in this field.
In AI-powered organizations like Google or eBay, AI is treated as a first-class citizen, not only in terms of product development but also in terms of data strategy and company culture. This means that the entire ecosystem, from product development processes to organizational culture, is centered around AI. This environment provides a fertile ground for aspiring AI PMs to learn, grow, and contribute significantly.
Network and collaborate
Building a professional network in the AI field can open doors to numerous opportunities and insights. Engage with AI communities both online and in-person. Platforms like LinkedIn and Slack allow you to connect with AI professionals and thought leaders, while forums and social media groups are great for staying updated on the latest AI trends and discussions. Attending AI conferences, webinars, and meetups can also be invaluable for learning from others’ experiences, sharing knowledge, and forming connections that could be vital for your career growth in AI Product Management.
Discover the World's Largest Product Management Community
Join Product School's private Slack community and network with over 100,000 PMs.
Join Now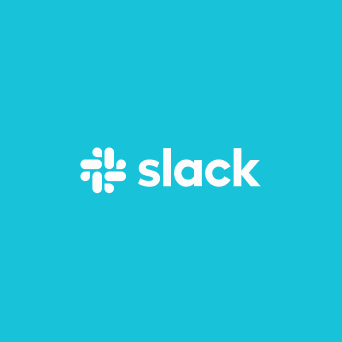
It’s time to lead the AI revolution
As AI continues to revolutionize the way we build and manage products, the role of Product Managers in AI-powered organizations becomes increasingly crucial. By understanding the AI product lifecycle, and mastering the necessary skills to overcome the unique challenges of AI Product Management, you can position yourself at the forefront of this exciting field.
Remember, in the world of AI, the possibilities are as limitless as your imagination. Let's embrace the future and lead the charge in shaping AI-powered organizations!
Updated: April 25, 2024