Updated: January 24, 2024 - 11 min read
Discover the impact of AI in product development where data becomes experience and algorithms turn into innovation. For PMs aspiring to become an AI Product Manager, mastering these technologies means far more than just engineering smarter products; it involves a deep alignment with genuine human needs.
Editorial note: This post is based on a talk by Joe Spisak, Meta Product Lead, on AI PM Through the Lens of Open Science and contains additional insights and examples from the Product School team. You can watch the webinar in full above.
In the role of an AI/ML PM, you'll find yourself at the vanguard, pushing the boundaries of traditional product management, demanding strategic thinking and critical decision-making at every stage of AI integration. Balancing the focus between technology and people, choosing between building in-house or buying, and developing a good data strategy are all part of the AI-enhanced PM journey.
In addition to the in-depth exploration of AI's intersection with product management, this post also teases additional insights:
The top considerations and insights for AI PMs
Actionable steps to dive deeper into AI and stay ahead
Tips to contribute to the AI and ML community
AI workflow: Engineering success in AI projects
AI workflow -from ideation to production. Let's deconstruct this workflow step by step, ensuring that your project doesn't just soar but also lands with precision.
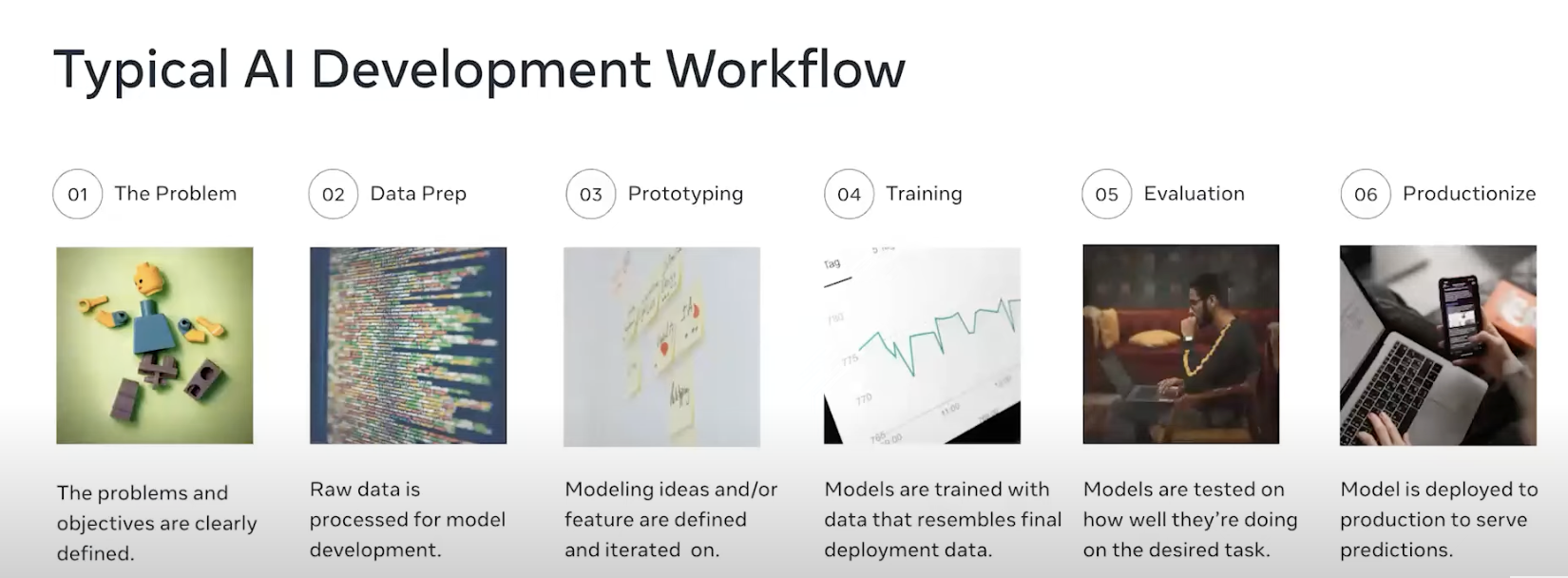
1. The problem: Defining the core of your AI initiative
As an AI Product Manager, you can’t afford to overlook the problem statement. It’s surprising how often this cornerstone is bypassed in the rush to leverage the latest AI model or dataset. Reflect - what exactly is the challenge you're aiming to tackle?
Imagine diving into a sea of data with a sophisticated AI model, only to realize you're swimming against the current of the actual problem. It's not only inefficient but can be a futile exercise. The key is to invert the process: start with a clear, precise problem definition.
What does this entail? You need to dissect the problem with precision:
Input analysis
What data or information will the model receive? Understand the variables, the context, and the environment of your inputs.
Desired output
Envision the ideal outcome. What form will the solution take? It could be a prediction, a classification, an action, or a recommendation.
Success criteria
How will you measure success? It isn't just about the accuracy of a model but the impact it has on the user experience, the bottom line, or the operational efficiency.
Learn more about Data in Product Management
Learn how data analytics can improve Product Management and make informed decisions with our Data-Driven Product Management: Using Analytics To Make Better Product Decisions post.
Read more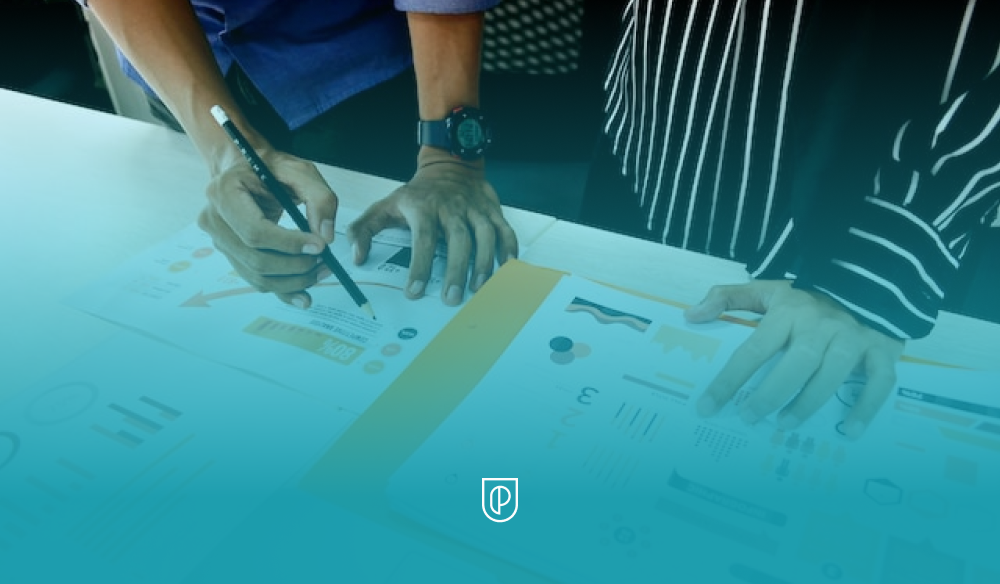
2. Data Prep: Curating your AI's knowledge base
Now, armed with a clear problem statement, it’s not just any data you’re after - you seek data that is relevant, quality-assured, and representative of the problem at hand.
Whether you're sourcing data from third-party providers, tapping into open datasets, or capturing new data streams, the imperative is to ensure that this data is fertile ground for your AI model to grow. The curation process involves several critical steps:
Annotation and labeling
Invest time and resources to label your data accurately. This could mean annotating images, tagging text, or marking up audio files. The precision of your labels equates to the clarity of the signals you send to your AI during its learning phase.
Cleansing and structuring
Roll up your sleeves for the painstaking task of cleaning and organizing the data. It involves correcting errors, dealing with missing values, and standardizing formats so that algorithms can process them effectively.
Debiasing
Vigilantly root out biases that could skew your model’s understanding. A biased model is a flawed model, likely to falter when faced with the real world's diversity.
3. Prototyping
To become an AI Product Manager, consider prototyping as a series of educated experiments, a sequence of hypotheses tested through the lens of data and algorithms. It’s here that you’ll get a first glimpse of the potential of your AI model. You don’t just jump to a complex neural network; you start with the basics, build up your understanding, and gradually increase the sophistication of your models.
4. Training - Where models gain muscle
You've explored the contours of your AI’s potential through prototyping, and now it’s time to hit the accelerator. This is where your model turns from a promising prospect into a performer.
Cost-benefit analysis
Prototyping becomes essential. It’s the due diligence phase where you ensure every hour spent in training is justified, every GPU cycle is a step towards a model that’s not just smarter, but also commercially viable.
Iterative excellence
Rare is the model that gets it right on the first pass. You’ll tweak and tune, refine, and repeat. This isn't a flaw in the process; it’s the process. No experienced PM expects a model to emerge fully formed.
The big picture
Remember, training is but one phase in the lifecycle of AI product development. Keep sight of the broader horizon — the deployment, the user experience, the problem you set out to solve.
Learn more about AI in Product Lifecycle Management
Discover the future of product innovation and unlock the secrets to revolutionizing the product lifecycle.
Read more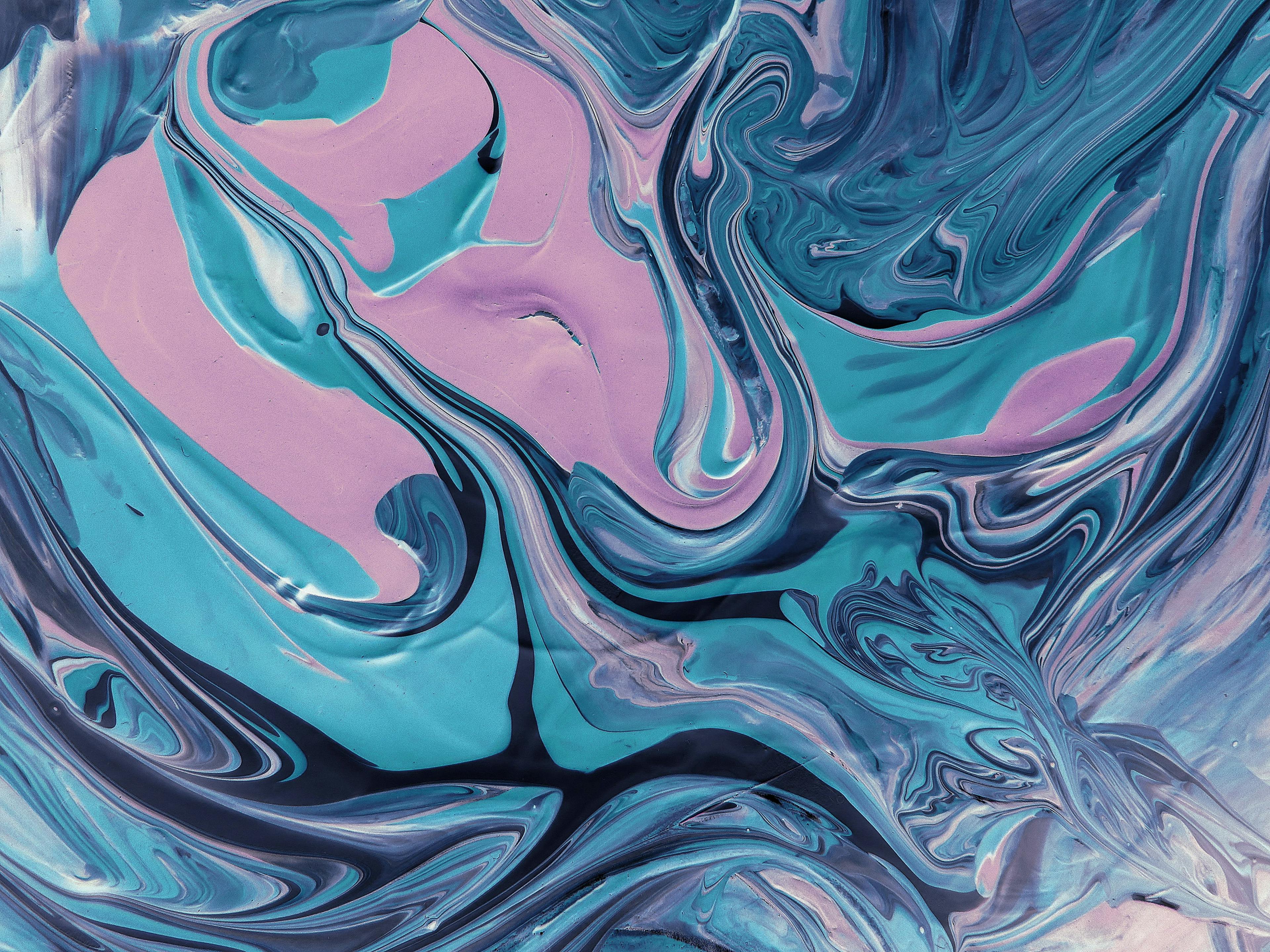
5. Evaluating your AI solution - The yardstick of success
Evaluating the effectiveness of your AI model is the yardstick against which the viability of your entire project is measured. This phase is where the rubber meets the road, providing clear, empirical evidence of how well your solution translates from theory into practical value.
Defining success metrics
Success in ML is quantified by well-defined metrics. As a PM, you must pinpoint what success looks like early on and make sure these benchmarks are transparent and aligned with your product goals. Is it about precision, recall, or perhaps the area under the ROC curve? These aren't just statistical terms; they're beacons guiding your product to its desired destination.
Actionable insights
Lastly, the evaluation should offer actionable insights. It's about understanding why it works or why it doesn't. This insight is vital for cross-functional teams to improve, iterate, and innovate. Evaluation illuminates the path forward, whether it's further data collection, additional training rounds, or a pivot in the product strategy.
6. Productionize: From model to market
You've shaped and honed your AI model; now it's time to set it into motion in the real world. Transitioning from development to production is where your solution truly earns its stripes.
The AI product manager's mandate
This phase requires a meticulous blend of foresight, strategy, and operational acumen. Production is not the finish line; it's a new beginning - a commitment to continuous nurturing, learning, and iterating.
The launchpad for growth
Deployment is just the start. Your model is now on a journey of constant learning from live data, customer interactions, and market feedback. It's an ongoing cycle of deployment, monitoring, adjusting, and enhancing.
How AI relates to PM roles
As PMs, you're no strangers to guiding a product from the seed of an idea to a fully-fledged feature in the hands of users. But when it comes to AI, your role isn't just enhanced—it's pivotal at every turn.
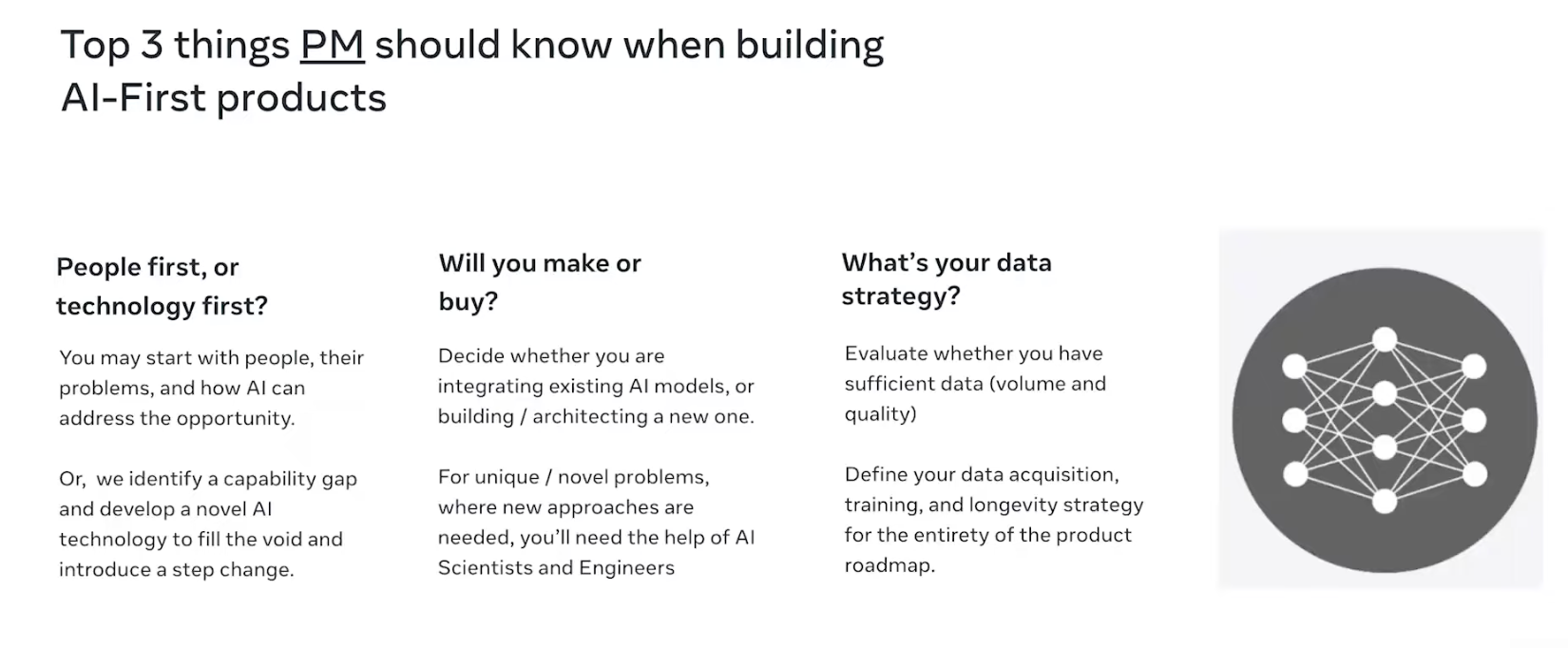
Top 3 considerations for AI Product Managers
It's your insightful inquiries and strategic thinking that pave the path for innovative solutions. To get the most out of AI, ask yourself these key questions:
1. Prioritize people or technology?
When venturing into AI-driven products, should we lean on technology as our starting point, or should user needs shape our technological pursuit? The answer isn't straightforward.
It might serve as a platform, sparking new applications that redefine user experiences. Alternatively, the classic approach of starting with a user-centric problem and seeking out technological solutions is equally compelling.
Balancing a people-first and technology-first mindset is essential. Both roads can lead to innovation — the key is to choose the path that aligns with your vision and goals.
2. Build or buy?
With the proliferation of advanced, off-the-shelf AI models, the opportunity to quickly prototype and test ideas is enticing. Within days, you could have a tangible proof of concept, accelerating your time to insight.
However, the decision to buy should be weighed against the unique needs of your project — is customization key? Or will an existing solution suffice?
Your role is to discern whether to invest in building a bespoke model or to adapt pre-existing technologies.
3. Devise a robust data strategy
Begin by asking: Do we have the data we need? Assess volume, variety, and velocity. Then, determine how you will acquire it — is it through partnerships, purchases, or in-house generation?
And let's not overlook the need for quality — how will you ensure the integrity and annotation of this data? Your data strategy must be comprehensive, considering not only acquisition but also management, privacy, and ethical implications.
3 Key insights for AI Product Managers
As you lead a cross-functional team, there are three pivotal insights you need to anchor in your strategy:
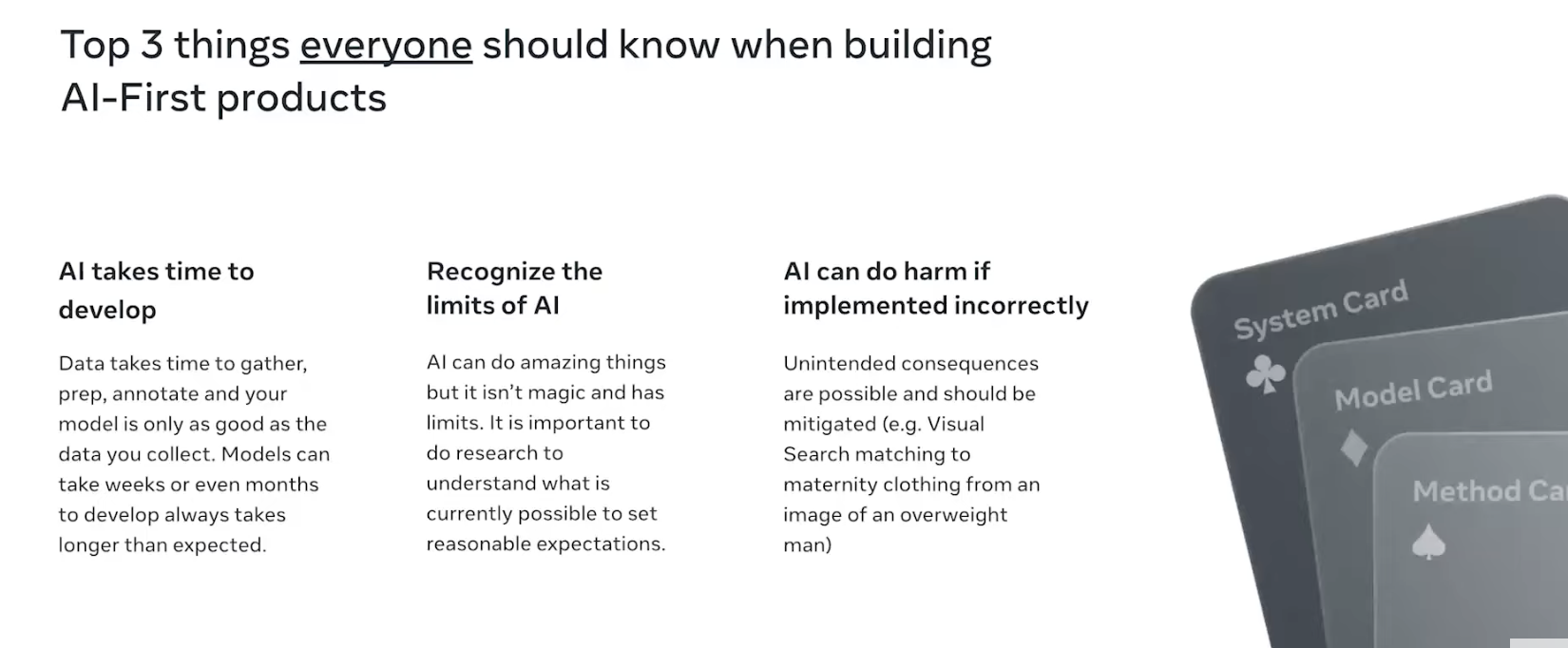
1. AI takes time to develop
AI is not an overnight sensation. Data collection, model training, and iteration unfold over weeks, sometimes months. That initial proof of concept is merely the starting line. Post-launch, you’re tasked with continual refinement, ensuring your model stands the test of time and use. Alongside this technical organization, layer business logic to safeguard against results that could undermine user trust or experience. Patience here is not just a virtue; it's a requirement.
2. AI has limitations
It's important to recognize that AI, for all its advancements, has its limits. As an AI Product Manager, your expertise isn't just in product roadmaps and user personas; it's also in understanding the cutting-edge capabilities—and limitations—of AI technologies.
This understanding guides you in setting feasible goals and managing stakeholder expectations. If your vision outpaces current AI capabilities, it may be wise to pivot or pace your product's evolution with the growth of the technology.
3. AI can harm if used incorrectly
AI comes with an imperative for responsibility. ML can cause unintended harm. This responsibility transcends the product—it's about the impact on society.
Your governance process must be meticulous, and your approach to deployment, thoughtful. You're not just optimizing for performance; you're striving for fairness, inclusivity, and safety. Geography, demographics, and diverse user contexts—each factor contributes to a complex ethical equation that you, as an AI Product Manager, must balance with care.
Action Items: Diving deeper and staying current
As a PM diving into AI, you'll require guidance, a map for strategic planning, and signals to keep you updated on the evolving technology environment. Here are some actionable steps you can take:
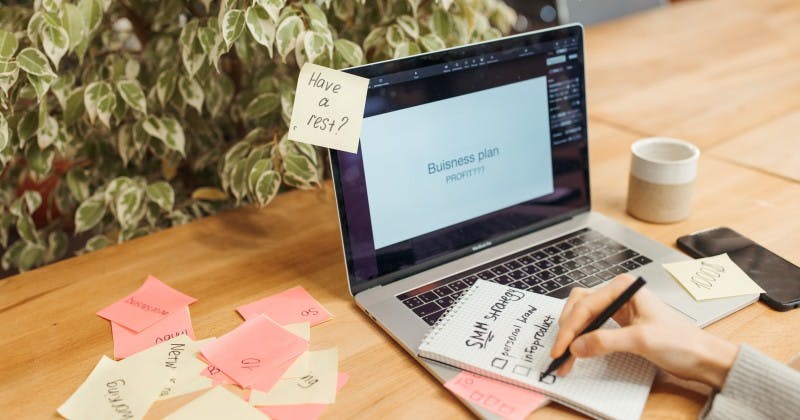
1. Follow the right people
Surround yourself with the luminaries of the field. Follow the trailblazers like Yann LeCun on social media platforms—his musings and insights can be as enlightening as his academic work.
Industry pioneers are a tweet away, disseminating knowledge, sparking discussions, and inspiring a whole new generation of AI enthusiasts.
2. Track innovations constantly
Your toolbox as a PM should always include a direct line to the pulse of AI research. PapersWithCode.com is a great resource.
This platform provides literature blueprints and building blocks of AI—datasets, code, and pre-trained models.
The methods section offers a behind-the-scenes look at AI, revealing how various algorithms synergize to produce the wonders of ML. Understanding these workings helps you enhance your knowledge and confidently guide your team's development efforts with innovative solutions.
3. Learn
Courses on platforms like Coursera can serve as both your foundation and scaffolding for higher learning.
These platforms aren't merely providers of information; they are incubators for the next generation of PMs in AI. They offer a space to learn, to question, and to grow. The key is to immerse yourself, to engage with the content and the community actively.
How you can get involved
Your involvement shapes not only your own products but the innovation. Here’s how you can carve out your niche and contribute to the ecosystem:
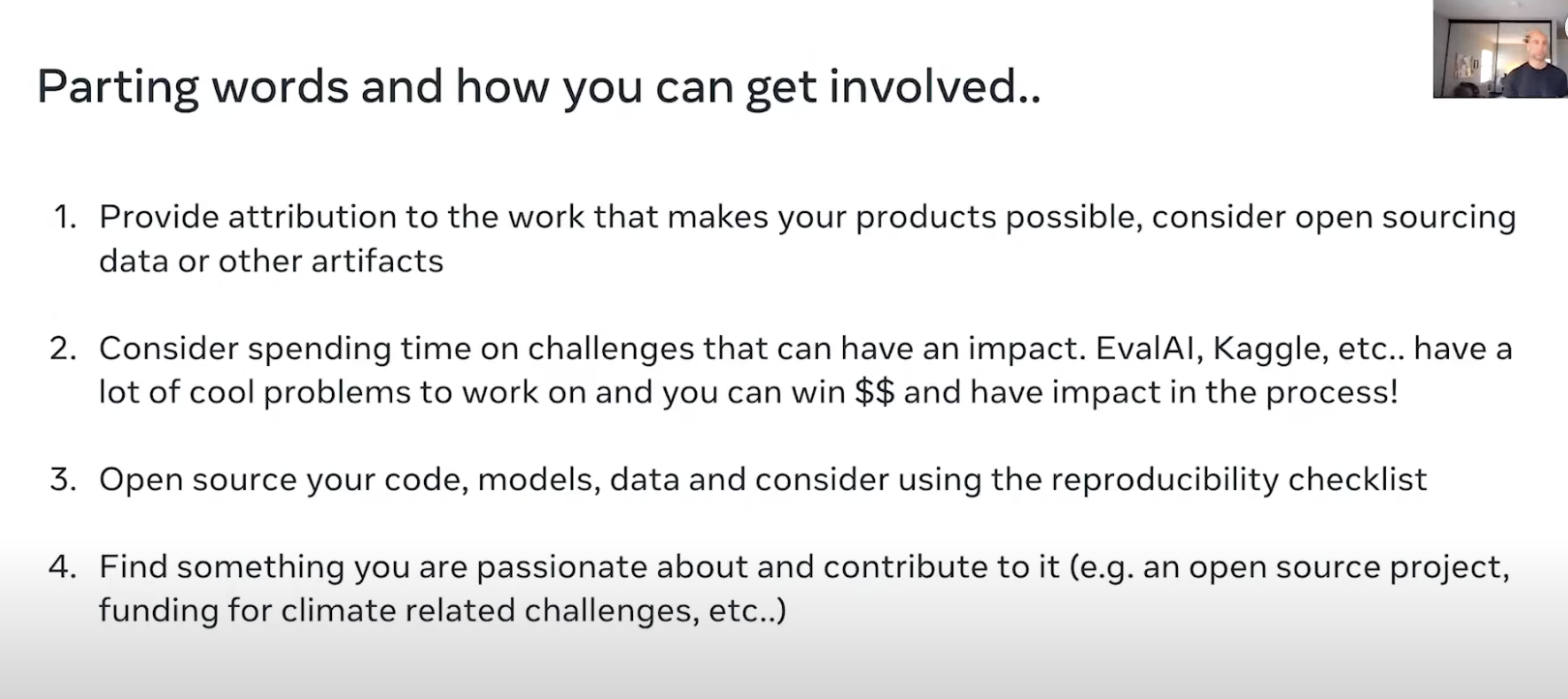
1. Provide attribution
Recognizing the collective intelligence that lifts your product is elemental. By crediting the researchers and acknowledging the open-source resources that contribute to your product's success, you demonstrate respect and shed light on the path for future innovators.
2. Spend time on challenges
As a PM, dedicate your skills to the pressing challenges within sectors like energy, supply chain sustainability, or environmental protection. Engaging in Kaggle challenges goes beyond competition; it's about applying product management principles to collaborative efforts aimed at solving critical global issues.
3. Open source your code
For those who are in research and development, consider the power of open-sourcing your work. Tools like reproducibility checklists not only enhance the credibility of your research but also invite collaboration. Sharing your code, models, and data, you'll be laying down the foundation for future innovation.
4. Find your passion and contribute
This industry thrives on contributions that extend beyond code. Your passion could lead you to write insightful tutorials that demystify complex algorithms or to enhance documentation making tools more accessible. Maybe your calling is to foster a community through meetups or virtual forums.
If you want to learn more about how to take the first step towards becoming an AI Product Manager, schedule a call with our admissions team today.
Updated: January 24, 2024