Updated: January 24, 2024- 11 min read
Everyone is talking about GenAI these days, but how can AI help you sift through massive datasets to make better product decisions? With the rise of big data, AI is a must-have in your Product Analytics flow. It’s like a data analyst intern, at super speed and super scope. You’ll have cleaner, faster, more accessible data insights at your fingertips – all the better to improve business and product outcomes with.
What is AI Product Analytics?
AI Product Analytics is the application of Artificial Intelligence to market and product data. Product Teams can use AI to automate data processes and analyze market and user data – including identifying patterns, delivering data insights, and making predictions of future trends.
Artificial Intelligence vs Augmented Intelligence
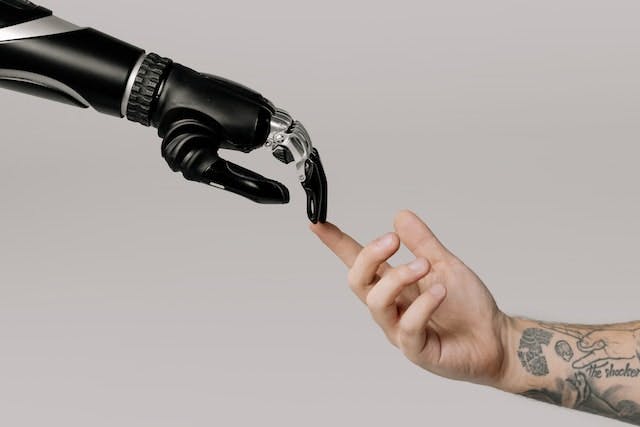
There’s a subtle distinction between artificial intelligence and augmented intelligence. Both drive efficiency, and both will play a part in the future of Product Management.
That said, the term “AI” is often used colloquially to refer to AU functions; in this article, we’ll do the same. But while we’re on the topic, let’s do a high-level overview on the difference between AI and AU.
Artificial intelligence (AI) is the use of machine intelligence to automate human processes and decisions, while augmented intelligence (AU) is the use of machine intelligence to support human processes and decisions. In other words, AI aims to replace human intelligence, while AU seeks to enhance it.
A type of AU you’ll see come up again and again in the data space is “augmented analytics.” Augmented analytics is data analytics run by machines and delivered to humans to support – or augment – their decisions and analysis.
Creating data-driven organizations at every level is one of the most significant trends in the data space; AI Product Analytics certainly lends itself to this. In the same way that no-code tools empowered non-technical teams to build and freed up engineering capacity, AI analytics will give everyone in the Product Team a key to peek into the black box of data. In fact, they can do more than peek in; they can walk away with armloads of insights.
This is the promise of AI – not to replace Product Managers, but to set them up for success. With the support of AI, you’ll have data to help you correctly identify customer problems, prioritize initiatives, and align your Product Team.
How to Use AI Product Analytics
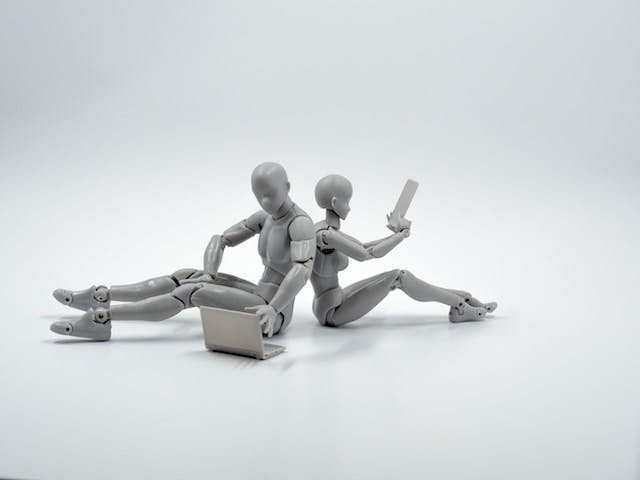
There’s a lot of hype around AI, but is it really better than traditional analytics? In a word… Yes!
Here are some ways AI Product Analytics can help you during discovery, launch, and beyond:
Problem identification
Idea validation
Usability testing
Flagging user behavior insights
Measuring product progress
Informing decisions for the future
Data presentation
Wait a minute… Aren’t those the same processes traditional Product Analytics helps with? Yes, well-spotted. But AI Product Analytics does them better, due to some standout functionalities of AI technology:
Data Process Automation
One of the best applications of AI in data is data collection, cleanup, and sorting. It seems basic, but quality datasets are the foundation of the rest of your analysis. Humans make lots of mistakes collecting data, and they take a lot of time to do it. With a bit of training, you can set up an AI model to collect data quickly and accurately. They can feed that higher-quality data to other, more advanced models that will use these datasets to improve their insights.
Adaptive Learning
You might get your self-improvement tips from LinkedIn influencers, but maybe you should start using AI as your model. AI algorithms are the masters of self-improvement (with the caveat that they are receiving good quality inputs, of course!).
AI analytics systems continuously learn and adapt over time, using machine learning algorithms to refine their models and predictions as new data becomes available. So not only does AI Product Analytics deliver better and faster insights than traditional analytics, it also delivers better insights than previous iterations of itself. How cool is that?
Traditional analytics, on the other hand, often require manual adjustments and updates. Not so cool.
Big Data Analysis
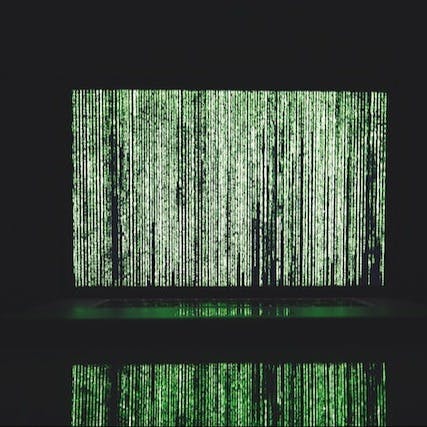
The ability to analyze more data = more scale in analysis.
AI Product Analytics can analyze big datasets with millions, billions, and even trillions of records. You can use AI to analyze information on lots of users, over long periods of time. Get insight into high-level user behavior trends and progress towards product KPIs!
Personalized Insights
You can also use AI Product Analytics to get more nuanced, specific insights. Data analysts only have so many hours in the day, and datasets are often underutilized – or worse, left unused. In other words, your team has been leaving meat on the bone at the data barbecue. AI is here to make sure you get every last byte.
AI Product Analytics tools can leverage advanced machine learning algorithms to identify correlations relationships within data that human analysts may overlook, either because these insights are not apparent or because they take too much effort to uncover.
AI can create granular user segments and recommendations in minutes based on its complex navigation of large datasets, something that might take an analyst hours to do. With AI Product Analytics, the future of personalization is looking good.
Unstructured Data Analysis
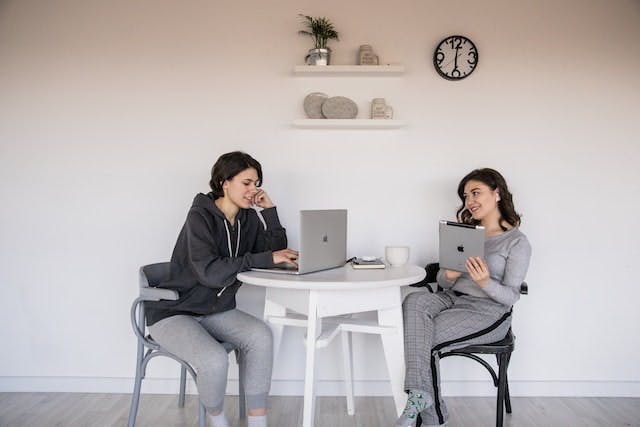
It’s time-consuming to analyze unstructured data with traditional Product Analytics. AI Product Analytics, on the other hand, tackles this kind of data with ease. AI models that depend on neural network technology – algorithms designed after human brain connections – are equipped to find meaning in user interviews and session recordings.
AI can quickly identify phrases and concepts that come up across the data and use them to provide recommendations and insights on things like user sentiment and pain points. This might just be the way you discover the next feature to build!
Predictive modeling
AI Product Analytics can’t read the future, but they can make a guess that’s pretty darn good. With advanced predictive modeling, AI can automatically draw predictions from the data and deliver them to Product Teams. Machine learning is designed to recognize patterns after all, and therefore excels at predicting next best steps.
Honorable mention: Market Research and Business Analytics
Though beyond the scope of Product Analytics, we’d be remiss not to mention market research and business analytics here. After all, the only way you have a product to analyze is because you were able to identify a need and build a business case for your solution. Product Managers can lean on AI to predict market trends, identify value-add opportunities, and model business scenarios.
Data Visualization
Data presentation doesn’t count as Product Analytics itself, but it deserves a shout. After all, this advanced analytics is pointless if you can’t use it to influence stakeholders! The way you present your data is almost as important as the data itself. Luckily, you don’t need to be a graphic design genius to get high quality data visualizations. You can make your data insights shine by using AI data visualization tools.
Is AI Product Analytics Right For Your Product Team?
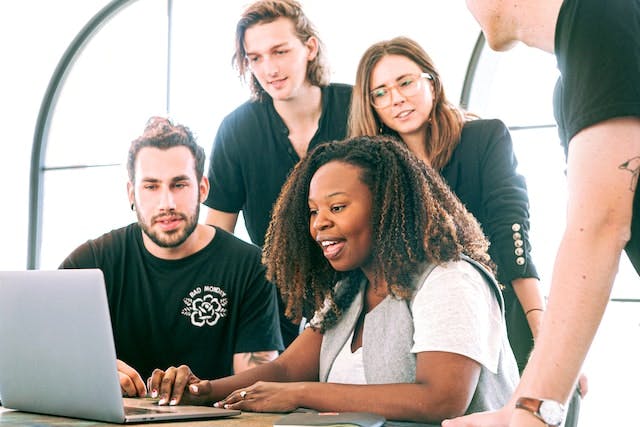
By now, you should be excited about the possibilities of AI Product Analytics. But before you go running to implement it, there are some factors you need to consider:
Data Security
Unless you work at FAANG or have an engineering team with the spare time to build in-house AI analytics tools, you’re probably going to be using external tools. If you collect user data and then pass it to another platform, you have to clearly understand how that platform is using the data. Even if it’s another company that misuses the data, it’s your responsibility to make sure nothing goes wrong – both for your users’ and your company’s sake.
This is true of any external tool, but it’s worth being extra attentive with AI. Because data is integrated into an algorithm, it’s more difficult to understand how the AI is using it. AI tools may inadvertently extract or infer sensitive information from user data that was not intended for analysis, or combine it with other datasets beyond original intent.
Do extra research into these third-party AI service providers to understand the risk. Ask questions. Is there a way for you to anonymize or de-identify user data before sharing it? Can the third-party use your user data in services designed for other clients? Does the company have privacy-by-design principles?
Cost
Who wouldn’t benefit from new, speedy AI analytics tools? Companies that would spending more than they’d be getting from these tools. AI is powerful and exciting, but it doesn’t mean it’s the right fit, right now for your company.
A large company with large datasets will probably get more value out of AI Product Analytics tools than they’re putting in. But a small company with a capable data analyst might be better off sticking with traditional, more cost-effective analytics tools than jumping on the AI bandwagon for the sake of it. Sometimes good is good enough! Run a cost-benefit analysis to understand: After all the increased efficiency and improved insights, is the investment worth it?
Early Adopter Syndrome
You’re here early! This is great news for you in the long-term because you’ll be way ahead of the competition. But in the present, this could spell trouble. Any new technology has bugs and unknowns, and AI Product Analytics tools are no exception.
There are great quality tools on the market, but they’ll change as the cutting edge of this technology evolves. Get ready to learn and relearn how to use the tools as they roll out improvements!
Over Reliance On AI Product Analytics
Remember: Augmented analytics is the name of the game, with emphasis on the “augmented.”
AI tools are still machines working from 1s and 0s. They’re only as good as the inputs they get, and can make mistakes or incorrect predictions. Your team still needs to apply critical thought to the reports they see. You’re the experts, and AI is the sidekick. If something in the data seems off, question it.
AI Product Analytics Tools
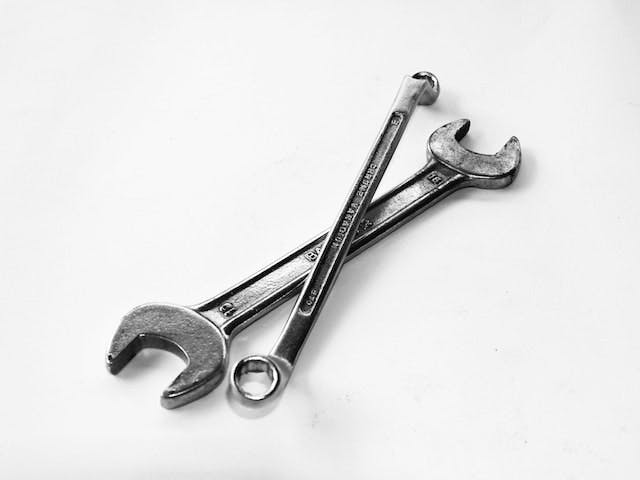
Hopefully we didn’t scare you away with all this talk of risks and constraints. Instead, we hope you feel confident and prepared to venture into the market with open eyes. To get you started, here are a few of our favorite AI Product Analytics solutions:
Tableau
A long-time industry front runner for data visualization and analysis, Tableau is now a leader in practical AI analytics applications (and it still particularly excels at data visualization in this space!). It offers no-code, augmented analytics for the whole team, as well as advanced modeling for data scientists.
It uses natural language processing to interpret user questions and delivers easy-to-read and interactive visualizations with the insights it uncovers.
ThoughtSpot
ThoughtSpot is an AI-powered data analytics and business intelligence platform with a self-service approach to data analytics. It’s a less-established player than Tableau, but was designed from the get-go with AI functionalities and is therefore slightly more optimized for this function.
It’s able to deliver high-quality insights quickly from large datasets through search-driven analytics, enabling less-technical team members to access insights. The platform's search capabilities extend beyond structured data and can also access unstructured and semi-structured data sources. It offers features like natural language processing (NLP) for intuitive querying, AI-driven data recommendations, and automated data modeling for simplified data preparation.
Polymer
Polymer is a powerful AI tool designed for data analysts, helping automate the process of turning data into a database – no coding required. The tool makes it easier for AI and human alike to analyze data in searchable and interactive spreadsheets.
MonkeyLearn
Ideal for analyzing speech or text, MonkeyLearn can help Product Teams clean, label, and visualize qualitative data from user interviews and feedback. Its data visualization capabilities are advanced and customizable and is ideal for running sentiment analysis and understanding user experience.
The Future of AI Product Analytics
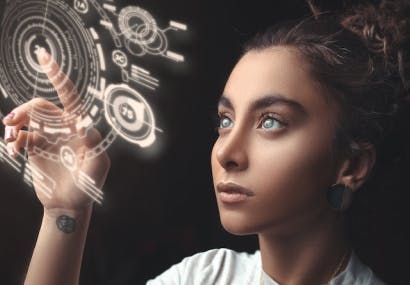
Imagine a world where data is optimized across industries and business types, from small shops to multinational corporations! As AI technology spreads and advances, it will become easier to use and applicable to edge-cases. Users will begin to have more control over datasets and applications, until every business and product has access to customizable AI analytics.
We’re not quite there yet, but that future is closer than it seems. Product Managers are always at the forefront in tech, and can pave a path for professions that will adopt AI analytics further in the future. Take this opportunity to lead the way!
Updated: January 24, 2024