Updated: May 6, 2024- 6 min read
You might be pondering how to integrate AI into your product teams and strategy. In this post, we delve into the best practices for product teams, offering insights to accelerate your journey to AI deployment.
Over 25% of investments in U.S.-based startups in 2023 are in AI-related ventures. This significant surge in funding speaks volumes about AI's potential. Companies like Synthesia and OpenAI have raised staggering amounts, underlining the immense value AI brings.
While integrating AI may pose challenges, understanding and addressing potential blockers is vital for success. You’ll also learn how to monetize AI-powered products, gain internal buy-in, and overcome limited developer resources. Even if you lack AI expertise, our guide offers solutions to leverage the expertise of third-party AI partners.
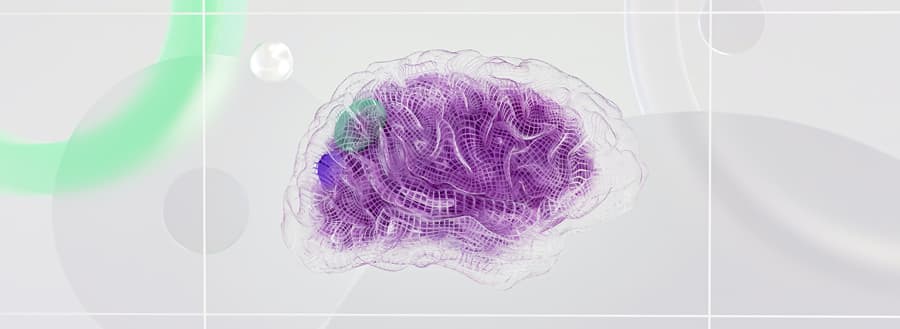
How to integrate AI into your product roadmap
1. Prioritize user value
Begin by assessing the value AI can bring to your customers. Define the specific problems AI can solve within your business context. What customer challenges can AI address? For instance, can embedding an AI model enhance process efficiency or help meet regulatory requirements?
Identifying user value is key to selecting the right AI model and optimizing its features effectively.
2. Set measurable goals
Once you've determined user value, establish measurable goals to ensure desired outcomes. For example, suppose you're developing an AI-driven recommendation engine for an e-commerce platform. You could set measurable goals like improving click-through rates (CTR) by 15% or increasing the average order value (AOV) by 10% through personalized product recommendations.
These goals would help you evaluate the effectiveness of your AI model in enhancing user engagement and boosting revenue. Ask yourself what outcomes your AI-powered product should deliver, whether it's better data accuracy, time savings, or customer benefits.
3. Develop a data management plan
Managing data is essential, whether you're building AI from scratch or customizing existing solutions. Collect and clean data aligned with your goals and capable of scaling with your AI product's usage. Consider data volume, sources, frequency, costs, privacy, and compliance issues. Decide whether to handle data internally or outsource based on your resources and expertise.
Key data considerations:
Frequency
Costs
Type
Source
Size
Privacy and compliance concerns
4. Choose the right AI model
Picking up the right AI model depends on user value, goals, and specific tasks. Consider whether to build in-house or partner with a third-party AI expert. In-house development offers customization but may require significant resources and expertise. Ensure you can support ongoing maintenance and infrastructure complexity. Collaborating with a third-party AI partner may be more feasible.
Popular AI Models for Business:
Content Moderation
Sentiment Analysis
Summarization
Face Recognition
Generative AI
Topic Detection
Fraud Detection
Entity Detection
PII Redaction
Diagnosis Prediction
Intent Recognition
5. Define Key Performance Indicators
Identify Key Performance Indicators (KPIs) to measure success, aligning them with measurable goals and chosen AI models.
6. Form cross-functional teams
AI product development needs collaboration among Engineering, Marketing, Product, and Support teams. Define roles and responsibilities for each team member. Cross-functional teams offer valuable feedback for enhancing customer experiences and adoption.
7. Ship, maintain, and iterate
After launching your AI-powered product, focus on long-term success. Manage user feedback, accommodate feature requests, monitor performance, and incorporate cutting-edge research.
Ensure your product continually utilizes state-of-the-art AI models. Consider whether internal research or partnering with a third-party AI research team best suits your needs. This ongoing process preserves your product's value and competitiveness.
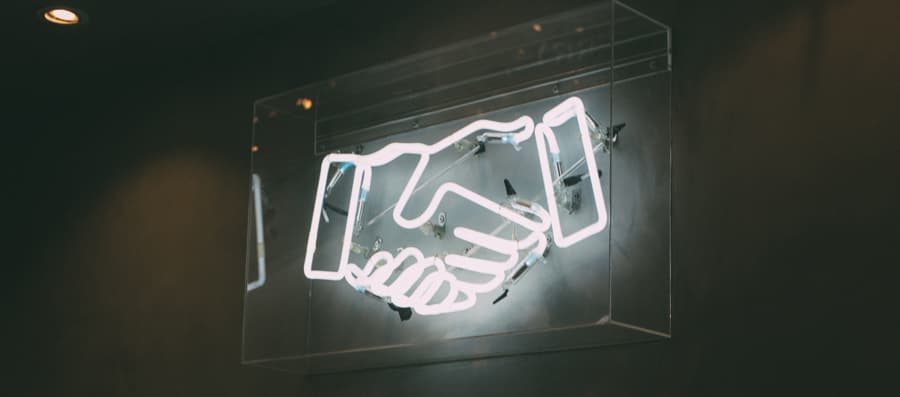
Choosing the right AI partner
Selecting the right AI partner is also a crucial decision when integrating AI into your products effectively. To ensure you make the best choice, consider these factors when evaluating potential AI partners:
1. Continuous AI model updates
Look for partners committed to continuous AI model updates. You want your partner to stay on the cutting edge. Ensure their models are regularly refreshed with new data and aligned with the latest AI research and advancements to keep your product competitive.
2. Strong in-house research team
A partner with a dedicated in-house AI research team is a significant advantage. This signals their expertise and commitment to excellence. Look for transparency through regular changelogs, showcasing their commitment to providing you with state-of-the-art models.
3. Ready-to-use AI models
Consider partners offering ready-to-use AI models that require minimal internal maintenance. With limited internal resources or a small engineering team, this option can save time and resources while still delivering the AI capabilities your product needs.
4. 24/7 support
Seek partners offering comprehensive 24/7 support, including health checks and personalized monitoring. This level of support is invaluable, especially for teams with limited in-house resources. It shows the partner's dedication to your success.
5. Transparency and security
Choose partners who are transparent about their data storage practices and openly share their research methodologies. Be wary of deceptive communication or misleading tactics. Ensuring advanced security provisions are in place is also crucial to protect sensitive data.
6. Pricing model alignment
Assess how well the partner's pricing model aligns with your business strategy. Consider factors like data processing frequency, scalability, and long-term profitability to make an informed decision.
7. Additional partnership opportunities
Look for AI partners who offer more than just technology. Seek out those willing to engage in collaborative efforts such as AI roundtables, early access to new models or updates, and co-marketing initiatives. A true partnership can accelerate your growth and provide strategic advantagesPotential blockers and risks
While the benefits are clear, understanding and mitigating these challenges is vital for success.
1. Difficult to monetize
For some product teams, the prospect of monetizing AI-powered products seemed daunting. It's understandable—without a clear path to monetization, it can be challenging to justify the investment. However, before throwing in the towel, consider revisiting the first two best practices we discussed: focusing on user value and setting measurable goals.
Ask yourself: How can an AI-powered product attract new users? Will it significantly boost revenue? Could it mitigate risks in infrastructure, data privacy, or compliance? Meticulously mapping out these aspects and continually assessing progress will pave the way for profitability.
2. Internal buy-in across multiple stakeholders
Ensure that your measurable goals align with your company's overarching objectives. This alignment makes it easier for every team to recognize the long-term value of AI integration, ultimately securing their support.
3. Limited developer and/or engineering resources
The concern of having limited internal developer and engineering resources is a valid one. Building an AI model from scratch in-house demands a substantial team of researchers and developers. However, there's a workaround—third-party partners that offer seamless AI model integration through APIs.
When seeking such a partner, prioritize those with a strong focus on ongoing research and development, coupled with on-demand support. This approach ensures access to top-tier models and a smooth integration process.
4. Lack of AI expertise
While a foundational understanding of AI models and applications is advantageous, don't let this obstacle deter you. Consider partnering with a third-party AI expert, as discussed earlier, to leverage their expertise as a solid foundation for your AI endeavors.
Integrating AI into your product teams requires a strategic approach. Despite the potential blockers and risks, addressing these challenges with a user-centric, goal-driven approach can lead to successful AI integration that benefits your organization and customers alike.
Updated: May 6, 2024