Updated: May 6, 2024 - 25 min read
Let's dive into the world of artificial intelligence (AI) in product management. Non-deterministic products are not the same as your everyday software, and that's precisely what makes them an exciting challenge!
If you’ve ever wondered about product management for AI or how to become an AI product manager, you’ve come to the right place. In this article, we’ll discuss the fundamental concepts that form the basis for AI products, AI product management, and what AI product managers do day-to-day. We’ll also share some tips for how to transition into the AI/ML product manager role including free resources and course recommendations.
Keep reading to future-proof your career!
AI PRD Template
Plan, strategize, and align stakeholders around the key requirements unique to AI products.
By sharing your email, you agree to our Privacy Policy and Terms of Service
Understanding Machine Learning in Product Management
First and foremost, let's demystify machine learning products. Unlike traditional software, where the outcome is generally deterministic and based on set algorithms, machine learning (ML) products evolve and learn from the data they're exposed to. It's a dynamic, ever-changing scene, and that means the product development process for ML products is equally dynamic.
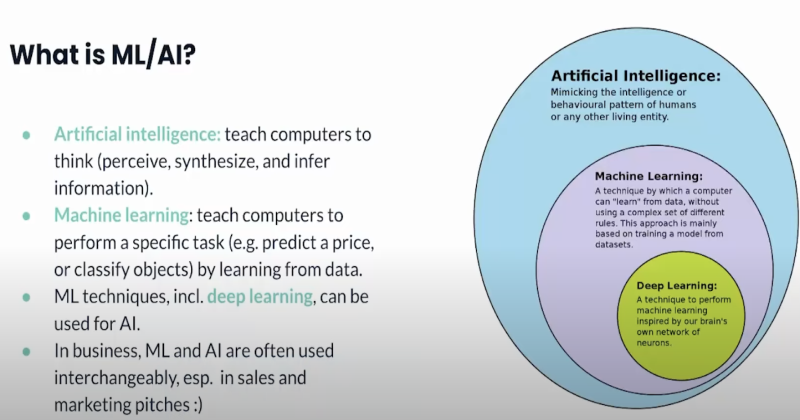
AI/ML product management: Terms and types of products
Terms like 'machine learning' (ML) and 'artificial intelligence' (AI) are often used interchangeably when in fact they are distinct terms. AI is the broader goal of machines mimicking human-like thought, while ML is a subset, teaching machines to learn from data.
On a scale measuring the role of ML in products, at one extreme, ML is the core, like OpenAI's GPT or Google's search. At the other, ML enhances the experience but isn't central, as with Amazon's product suggestions or Netflix recommendations. In between, products like virtual assistants or self-driving cars rely heavily on ML but not exclusively.
What sets ML products apart?
ML products carry a promise, a charm, a challenge, and sometimes, a headache for product managers. So, what defines machine learning in product management?
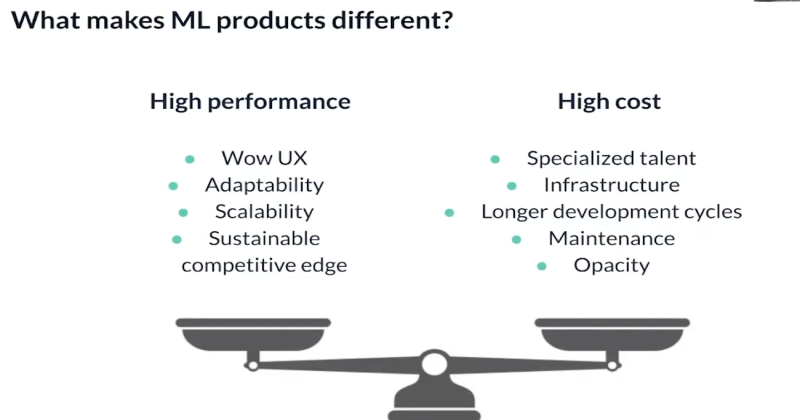
The allure of high-performance
High performance is the crowning jewel of ML products. It’s what justifies the high costs that often accompany them. These should include:
1. The "Wow" element
The pinnacle of ML achievement is that jaw-dropping user experience – an outcome so impressive that it can't be replicated with mere rule-writing or arbitrary calculations. It's that 'magic' that leaves your users spellbound.
2. The art of adaptability
ML's beauty lies in its adaptability. Whether it's evolving user behavior or changing environmental conditions, a well-designed ML product seamlessly adapts, ensuring relevancy and precision.
3. Scalability at its best
Starting with one user segment and expanding to others? ML products, when adequately resourced, can scale gracefully, meeting varied needs without skipping a beat.
4. A lasting competitive edge
Nail your AI/ML product, and you’ve not only created a marvel for now but have also equipped your business with a sustainable competitive advantage. It’s a skill that, once mastered, can be leveraged across multiple challenges with spectacular results.
The price tag: high costs
However, the splendor of high performance doesn’t come cheap. Here's the price you pay for the AI extravaganza:
1. The need for specialized talent
You can't merely swap roles here. Machine learning engineers are a unique tribe, specialized in their craft. If you bring them on board, ensure you've got a rich pipeline of projects to keep them engaged and productive. The lack of AI/ML product managers, developers, and UX specialists means that it’s hard to keep up with demand.
2. Robust infrastructure
Planning to roll out complex models at scale? Brace yourself for significant investments in computational prowess and data storage. These aren’t your regular tech stacks.
3. Patience-testing development cycles
If you're accustomed to rapid, incremental progress, AI products often longer development cycles can test your patience. But remember, the wait is often worth the marvel that emerges.
4. The challenge of opacity
Transparency isn't ML's strongest suit. The 'black box' nature of some ML models can be a tad unsettling for those unfamiliar with its intricacies.
5. The demands of maintenance
ML products aren’t set-and-forget. From ensuring real-time data feed for accurate predictions, to continuous monitoring and fine-tuning, the maintenance legwork is intensive. It demands resources, attention, and, above all, a commitment to excellence.
Level up on your AI knowledge
Take a deep dive into the world of AI with Product School's definitive guide.
Download Guide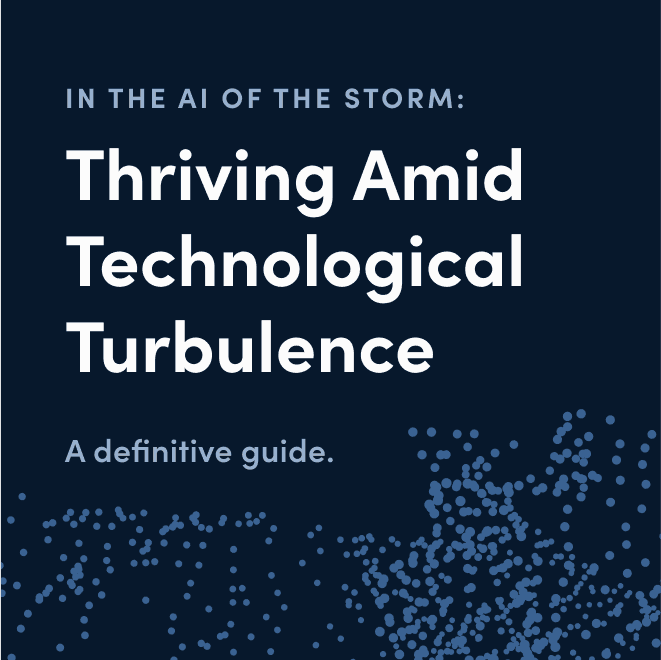
Developing and Delivering ML Products: The Foundations of AI for Product Managers
These are the key components you'll need to make it happen. Machine learning, while fascinating, does require a blend of resources to bring models to life. Two primary components form the bedrock of machine learning: data and algorithms. Think of them as the twin pillars supporting the edifice of your ML journey.
Data: Machine learning needs data, much like a car needs fuel. AI PMs need to be able to understand data in the form of structured information tailored to their prediction target, along with relevant features aiding the prediction. For predicting search result clicks, the clicks are 'labels' while user behavior and search details are 'features'. An example dataset is the Boston Housing dataset, where house prices are the labels and house attributes are the features. This helps test different models.
Algorithm: The algorithm turns data into actionable insights. Its complexity can vary, from simple linear regression to complex neural networks. The best choice depends on the specific task. Whether it's linear regression, decision trees, or neural networks, selecting the right algorithm is crucial.
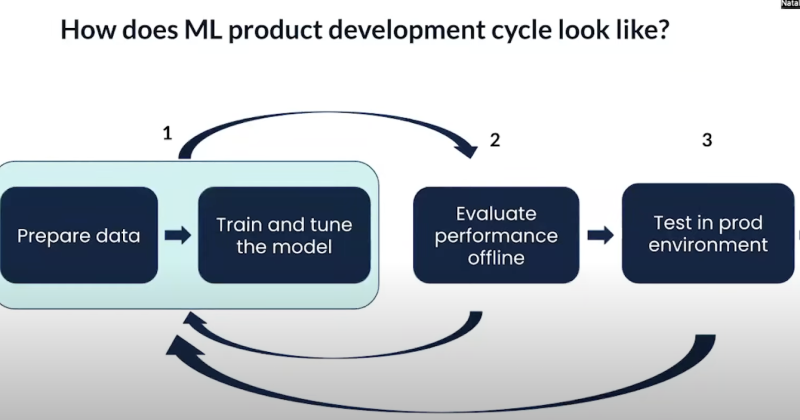
ML/AI product development goes beyond coding. It involves model training, data selection, and ongoing refinement. As a product manager, you'll navigate challenges unique to this field, coordinating with data scientists and ensuring algorithms meet user needs.
Let's dissect this journey, step by step, for clarity.
Model training
At the very onset, you're aiming to train a potential model. This process has two key components:
Data preparation: More than just cleaning data, this step involves strategically crafting the key features for model training. It's a blend of science and creativity and is fundamental to ML projects.
Algorithm selection & tuning: After preparing your data, you'll select and then fine-tune the right algorithm. While the details are technical, understand that optimizing your algorithm greatly enhances your model's effectiveness.
Performance evaluation
Once your model is trained, evaluate its accuracy. Regardless of its task, your model should get better with each iteration. After confirming its offline performance, you can move to real-world testing.
Real-world testing
A/B testing compares your model to a control group or a previous version, assessing its impact on key business metrics. If metrics meet your goals, you can move the model to production. However, success may require multiple iterations and adjustments. Although you hope for consistency between offline and online results, discrepancies can occur due to technical issues, UI changes, or other challenges. If results differ from expectations, use a checklist to pinpoint potential problems.
Common challenges for AI/ML PMs
Poor data quality and availability: Despite data abundance, the quality and availability of data remain a significant challenge. The data collected through legacy systems often doesn't align with the needs of modern AI/ML systems.
Data collection and cleaning: As an AI/ML PM, you're responsible for ensuring that the data collected is as clean and relevant as possible. This means not just collecting data for the sake of it but focusing on gathering data that adds value to your product.
Addressing data-related challenges: This includes evaluating data sources for quality and relevance, implementing data cleaning processes, and developing a strategic data collection approach. Collaborating with data engineers and scientists is essential to align these practices with the broader goals of AI/ML development.
Essential Skills for AI Product Managers
The AI product manager job description is as varied as a traditional PMs. Each day comes with its own challenges, many of which depend on the type of products and features that are in the pipeline. However, there are certain skills that are unique to AI/ML product managers, including:
0. Understanding the tech
To be effective in directing and guiding your team, you should grasp the basics of how algorithms work, the data requirements, and the challenges in training and deploying models. The clearer you are about the mechanics of ML, the better you can steer the product direction, set realistic expectations, and handle stakeholder interactions
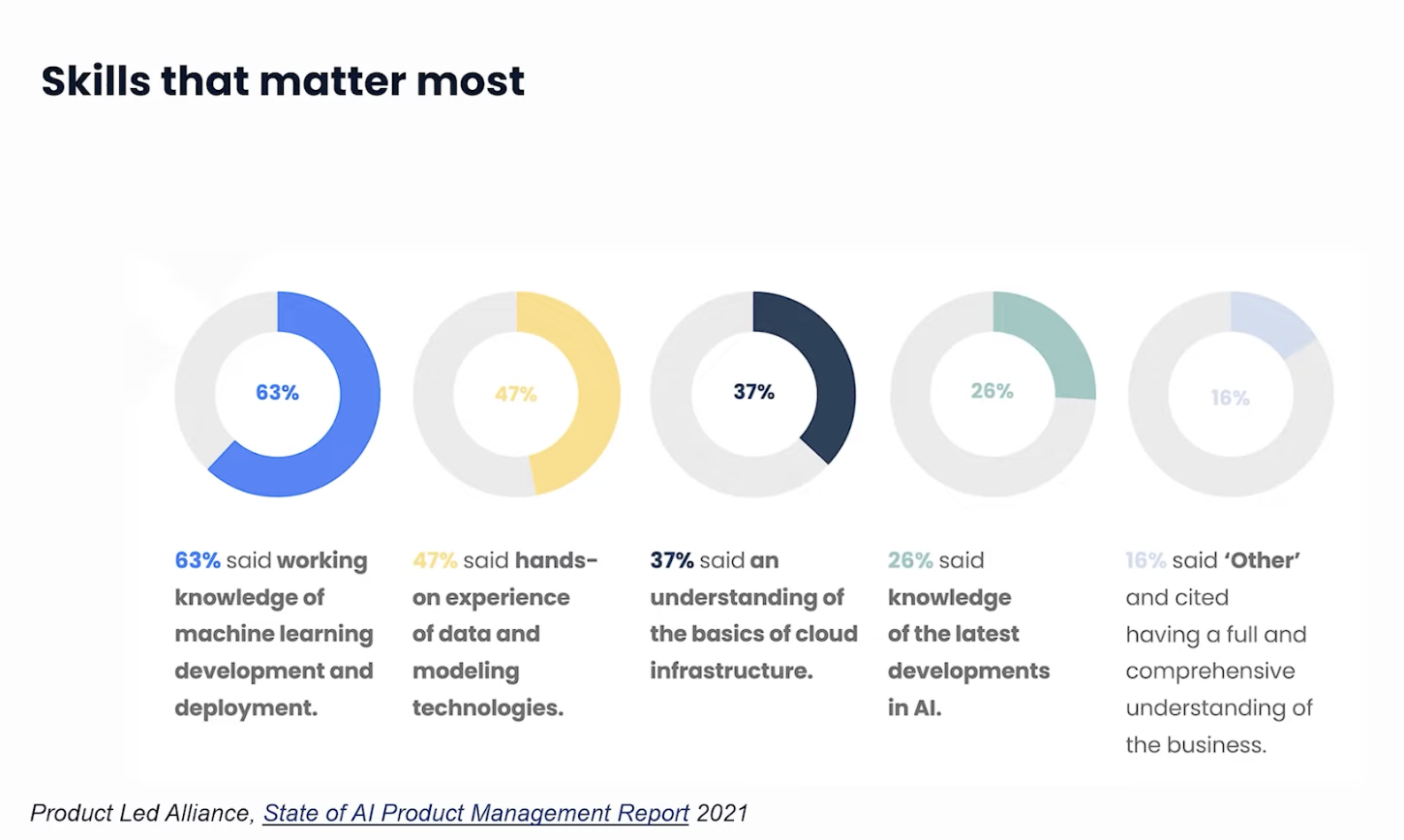
1. How to define the problem and business opportunity
In the process of solving a business problem with Artificial Intelligence (AI) or Machine Learning (ML), the first step is to determine whether an ML or AI algorithm is the right approach to address the problem.
Machine learning algorithms excel at uncovering complex relationships and hidden patterns in datasets that consist of many interdependent variables. However, it's vital to acknowledge that machine learning and AI is not a universal solution for every problem.
Some cases can be resolved effectively without resorting to ML techniques, particularly when the target value can be determined using simple rules, computations, or predetermined steps that don’t require data-driven learning.
2. How to leverage the right data
When solving business problems with AI and machine learning, we encounter various challenges. The first challenge revolves around data accessibility. Do you have access to the data you need?
Sometimes, the problem may not be the absence of data but rather its inaccessibility. When dealing with large companies, data availability can pose a significant hurdle. You might have ample data for customer information, but accessibility issues could prevent your model from utilizing it effectively.
Another critical consideration is whether you should use the available data at all. You might possess sensitive customer information, including details about their residences and bank accounts. The question is, should you leverage this data? A good rule of thumb is to ask yourself whether you'd want to be in the news for using this data. If the answer is no, it's advisable not to use that information.
While your intentions may be to enhance the customer experience, customers may not share the same sentiment, and respecting user privacy is paramount. Ensuring that the entire data pipeline is secure is essential to prevent any data compromises.
Data relevance is another challenge. Relevant and up-to-date data is crucial for accurate predictions, but not all data at your disposal may be pertinent to the specific problem you're attempting to solve.
3. How to address bias
Addressing bias in data is another one of the critical skills for AI Product Managers. Bias occurs when machine learning algorithms produce anomalous outputs due to assumptions made during algorithmic development or biases in the training data.
Consider a scenario where you build a model to prioritize resumés for interview selection. While this problem seems suitable for machine learning, you may have a biased dataset, as it only includes data from candidates who applied and progressed through the process. This omission introduces bias because you lack data from potentially qualified individuals who never applied. Ensuring a balanced dataset is essential to mitigate bias and improve model accuracy.
4. How to analyse user feedback
In the context of machine learning, gathering customer feedback becomes even more crucial due to the probabilistic nature of machine learning systems. Probabilistic systems inherently require more data to enhance the quality of their outputs over time. The challenge lies in how to effectively collect this valuable data and create customer feedback loops.
One effective approach to gathering feedback is by embedding a feedback mechanism directly into the core customer workflow. To illustrate this, let's consider the example of Uber. Uber prompts its customers for feedback every time they log into the app. By doing so, Uber can accumulate a wealth of valuable customer signals. For instance, if a customer expresses dissatisfaction with the number of pickups, Uber can use this feedback to optimize its pickup algorithm. This approach exemplifies how incorporating feedback loops into the customer experience can lead to continuous improvement and enhancement of the user experience.
5. How to apply UX best practices to AI products
“On the bus, everyone gets the same stops - the same predetermined experience. In a taxi, each user puts in their own destination, and the driver creates a personalized journey for them. Similarly, products will evolve from being deterministic as they are today, to non-deterministic, allowing for a range of different experiences for every user. ”
– Sam Stevens, AI Product Leader & CEO at CatalistAI. Ex-Google, YouTube, Tinder
When it comes to user experience in product management, we can categorize it into conscious and unconscious elements.
Conscious user experience refers to situations where users actively understand and interact with the interface in front of them. For example, users might be asked to select from a dropdown menu, click one of two buttons, or choose between different color options. In these cases, users consciously make decisions based on the provided interfaces.
However, there's another dimension to user experience, which is unconscious. In unconscious user experiences, users may make decisions without consciously realizing it. An example of this is when a specific option is highlighted more prominently than others, and users unconsciously prefer that option. Many AI systems influence user experiences in unconscious ways.
A practical illustration of this concept can be seen in search engines. Consider two users searching for the same word, "shoes." While one user is shown men's shoes, another is shown women's shoes. This difference is due to the AI tailoring the results based on the user's gender information, creating an unconscious experience change.
As a product manager, you're leveraging user information to enhance their experience without requiring explicit input. However, it's crucial to consider what information you use to improve the customer experience and be mindful of potential privacy concerns and ethical considerations.
Another key aspect of designing user experiences with AI is to be opinionated. There can be multiple correct answers to a single user query. For instance, if a user searches for "couch," should the results display home decor ideas or different couch brands? The choice depends on the desired user action. If you're a retailer like Bed Bath & Beyond, you might prefer showing home decor ideas to encourage users to explore various products. In contrast, a search engine like Google may prioritize showing different couch brands to deliver more precise results.
To guide user actions effectively, you must design your interface to nudge users in the direction you want them to take. This involves simplifying the user interface and ensuring there are at most one or two clear actions for users to follow. By being opinionated and aligning the interface with your desired outcomes, you can enhance the user experience and achieve your product's objectives.
AI User Flow Template
Essential steps applied to real-world examples from AI product management break down a complicated process into clearly defined flows from the initial entry point to the generated output.
DOWNLOAD FOR FREE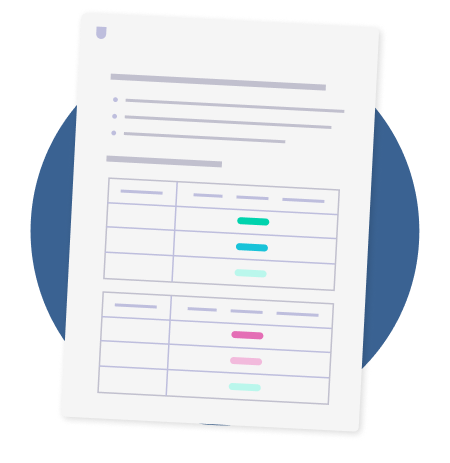
6. Effective collaboration with cross-functional teams
As a PM, you’re already accustomed to working with multiple stakeholders, but when dealing with AI products, this complexity increases significantly. In a traditional product management lifecycle, you collaborate with engineering, UX, customer success, marketing, and possibly sales teams to define requirements and iterate to build and ship the product.
When you're working on AI products, numerous new teams come into play. These teams may include data scientists, data engineers, machine learning scientists, machine learning engineers, applied scientists, and business intelligence professionals. This influx of stakeholders adds complexity to the product development process, as coordination and communication demands increase. The coordination time with these teams rises significantly, impacting the time required for product iterations.
In AI product development, if you receive feedback that necessitates changes to the AI models, the process can reset. This involves revisiting data requirements, addressing missing data, model building by the science team, and collaborating again with engineering for deployment. Therefore, more stakeholders, increased complexity, and longer iteration cycles are characteristic of AI product development.
7. How to monitor regulations
The AI and machine learning field is influenced by both technology and regulations. As product managers, stay updated on current rules and upcoming changes. These guidelines affect your data access, model deployment criteria, and management methods.
Stay informed
In the AI realm, regulatory knowledge is crucial. Stay updated on current guidelines and upcoming changes, as privacy laws and data access can significantly influence your AI product strategy. Being informed ensures not only compliance but also a product that respects user privacy and meets all standards.
Prioritize compliance
In the evolving AI field, strict regulations stem from past mistakes. See them as a chance to create a trusted product. By anticipating and complying with these rules, you instill trust in your product, ensuring smoother regulator interactions and boosting user confidence.
Know your regulations
Different regions have distinct data privacy rules, such as Europe's GDPR or California's Consumer Privacy Act. In domains like online advertising or e-commerce, understanding antitrust laws is crucial. Additionally, as AI-generated content grows, copyright concerns arise, especially when AI models reproduce artworks without permission. These discussions highlight potential challenges for artists and the risk of AI overshadowing human creations. In your AI journey, view regulations as guiding principles, not just obstacles. Your task is more than just adhering to rules; it's about ensuring best practices, protecting users, and building a lasting, trustworthy product.
8. How to plan for scalability
In the dynamic digital world, scalability is essential. With machine learning, you're not just addressing today's needs but also preparing for tomorrow's demands. Your role ensures growth, adaptability, and future readiness.
Optimize existing solutions
Review your current model before seeking new solutions. Can it be refined or adapted for other tasks? Given the effort and resources put into creating a model, it's essential to utilize it fully. This approach optimizes returns and broadens your impact with one investment.
Future-proofing with foresight
Look ahead and anticipate future challenges. How can your current resources address them? As a product manager, connect the present to the future. By proactively spotting issues and aligning them with your team's strengths, you can boost efficiency and ROI.
Embrace modular and flexible design
Scalability hinges on adaptability. By adopting a modular and flexible design, you can modify or scale your solution without a full redo. This conserves resources and quickens adjustments to evolving situations.
9. How to integrate AI into existing products
How and where will the model's predictions be displayed? How do you turn these outcomes into actionable results for users? Whether it’s enhancing existing features, enabling new features, or creating new products, understanding the integration path is paramount.
Enhancing existing features: Refining a recommendation system to align more closely with user preferences.
Enabling new features: For example, Google's reverse image search represents a leap in search technology.
Creating new products: For instance, developing enterprise tools that provide insights into organizational patterns and trends.
Applied AI Product Skills: Couples Therapy Case study
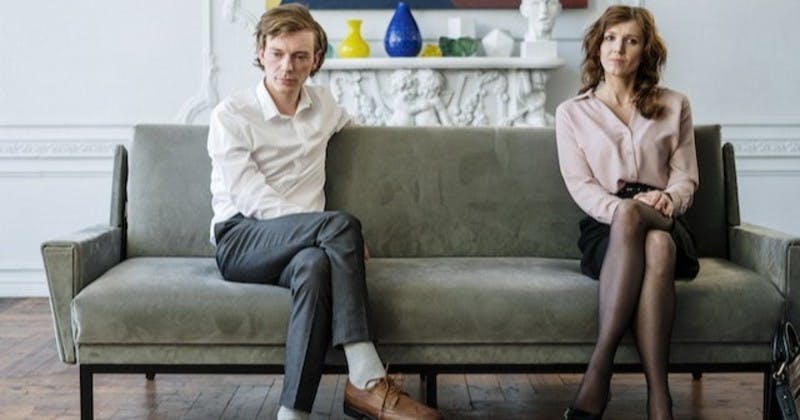
Let’s explore the application of generative AI in couples therapy. With Large Language Models (LLMs) taking centre stage in today’s media, it’s an intriguing question to ask - can I make a product that can replace my expensive couples therapist?
Editorial note: these ideas were originally published in a guest blog post by Ankit Srivastava
1. Start with the Problem - Not the solution
Just as with any other Product Management role, an AI Product Manager follows the same paradigm: your primary responsibility is to identify the customer’s pain points.
Technologies such as large learning models (LLMs), machine learning, artificial intelligence, and blockchain are merely tools to address these problems.
However, the flip side of AI's capabilities is that it can now uncover and solve problems that may not have been apparent before. This highlights the importance of thoroughly understanding the magnitude of the problem and assessing whether solving it would lead to growth opportunities. In other words, conducting customer research becomes even more crucial. By engaging with customers, gaining insights into their needs, and evaluating the potential impact of addressing those needs, you can make informed decisions about which problems to prioritize and how to leverage AI effectively. Remember, customer research plays a vital role in aligning AI solutions with market demand and maximising the growth potential of your products.
For the couples therapy example, I did a quick user study spread across 15 couples at various stages of their relationship. I asked each couple about 20 questions. What did I learn? Most couples are spending a considerable amount of time and money on couples counseling. While almost all of them find counseling useful, very few of them find it affordable even with insurance covering part of the costs. So the next question we need to ask ourselves is: Can we make a solution that is readily available, affordable and just as good as a counselor? Do we think AI, and LLMs more specifically, can be used to solve this problem? I’ve tried to answer this question in the next few steps.
2. Do I have the necessary Data?
Successful Product Management for AI revolves around identifying and utilizing the right data to create innovative products that captivate customers and foster long-term engagement. It's important to consider the strategy of sharing Product data with large-scale cloud providers when utilizing public large learning models (LLMs), as data can serve as a competitive advantage and sharing it may lead to a disadvantage in certain cases.
Many AI capabilities often lack labeled or annotated data, making it crucial to prioritize items based on data availability. For areas that require more annotated data, it's essential to devise strategies that allow for obtaining additional annotated data or leveraging publicly available data partially.
Furthermore, it's vital to have a clear understanding of how and if customer data will be used for training models. This involves anonymizing the data and obtaining explicit customer consent.
Now, let’s go back to our couples counseling app. Here are some important considerations to think about before saying that you can build an AI solution:
Is there any data related to couples counselling that can be used to train a model? In this case, the answer might be yes.
Can data be collected from customers to enhance model efficiency? It's worth noting that most customers may be hesitant to release their personal data to an online service.
Looking at the answers above, one might strategize to use AI and develop a private LLM model that does not share data and only uses anonymized customer data for training.
By addressing these questions and taking appropriate measures, Product Managers can navigate the challenges of data availability, privacy, and consent while leveraging AI.
Now let’s say you’ve worked with your development and user experience team and made a generative AI solution that can partially replace a therapist. The next question you should ask is:
3. How do I measure success?
This is an important question you'll face and is relevant for you to answer for the success of your product. However, measuring the success of AI features requires a different approach compared to traditional metrics like CSAT, PS scores, and CTR values. While measuring performance of a model using Precision, Recall and F1 scores is important, there are additional metrics especially in the case of LLMs which are also important.
Here are some additional metrics that I think are helpful to know as an AI Product Manager:
1. Hallucination Metrics
For this, analyze the generated output and compare it against the ground truth or reference data. Identify instances where the generated output contains information that is not present in the ground truth.
So for our example – For a given couples counseling question, define certain entities that are required in the answer for it to hold true.
Now measure times when none of these entities show up in the final answer given by the LLM. This will tell you when the model is mostly making up answers.
2. Semantic Similarity against human-generated answers
Measuring semantic similarity in AI models involves assessing the degree of similarity or relatedness between two pieces of text. In this case this would measure text between output generated by LLMs as compared to what a human would have responded with.
This helps us understand how close the answer was to a human response.
3. Latency (p75,p90,p99)
Latency is an important metric for assessing the efficiency and responsiveness of AI models, especially in real-time or time-sensitive applications. As a Product Manager, you will need to make sure your models respond fast enough or else most customers will lose interest.
Remember every feature developed, including AI features, should align with larger end goals. Whether it's driving usage, revenue, downloads, or other metrics, Product Managers must always connect their efforts to tangible outcomes.
Career Paths: How to Become an AI Product Manager
Eager to venture into ML/AI product management? The route isn't set in stone. Whether you're tech-savvy, data-oriented, or business-driven, there's room for you. To begin, dive into data, grasp AI/ML basics, partner with data scientists, and prioritize user needs. Whether pivoting as an experienced PM or starting fresh, the ML/AI realm promises endless opportunities.
1. Immerse yourself in an ML-driven company
To truly grasp ML, immerse yourself in an innovative company. Leading ML companies offer rich learning experiences and mentorship. Begin as a PM, show interest in ML, and soon you'll be collaborating with data scientists and shaping AI advancements. This setting not only boosts your ML expertise but also hones essential soft skills for the domain.
2. Advocate for ML within your current role
Why seek externally when you can pioneer internally? If your current role doesn't involve ML, introduce it. Find areas where ML can enhance value, research thoroughly, and pitch with conviction. This not only highlights your leadership but also positions you as the company's ML expert. Your initiative might spur new projects or even spawn dedicated ML teams.
3. Forge your own path with an ML startup
If you're entrepreneurial and see an ML solution to a unique problem, consider starting a venture. Assemble a team and create from the ground up. This challenging route provides deep insights into ML, business strategy, and fundraising. Leading your own startup gives you the chance to directly influence its trajectory and leave a lasting impact in the ML realm.
Whichever path you choose, remember that the world of ML is vast and ever-evolving. Continuous learning, curiosity, and adaptability are your best allies. Dive in, embrace the challenges, and watch as you transform from a product manager into an ML Product Management maestro. Your journey begins now!
4. Learn how to master AI products from an experienced instructor
Breaking into AI Product Management requires a unique set of skills, focusing on generative AI, data-driven decision-making, and user experience innovation. It goes without saying that artificial intelligence is a complex topic to understand, let alone apply to AI/ML products. Honing your skills through a course or certification is a great way to set yourself up in the world of AI product management.
Get acquainted with the foundational concepts through a free micro-certification or specialize under the tutelage of an experienced AI product expert with Product School’s Artificial Intelligence Product Certification (AIPC)™.
Hear from the first ever AIPC™ cohort
AIPC™ is here to transform you into an AI Product Manager. Master AI integration, AI product design, and more to elevate your PM career with AI expertise.
Read moreAIPC™ is meticulously designed to empower you with these skills and help you level up as an AI Product Manager. Discover AI fundamentals, build cutting-edge AI products, craft superior user experiences, optimize product performance using AI, and much more. Our certification is your gateway to becoming an AI Product Manager.
During AIPC™, students have a chance to build a low-code LLM-powered app, and everyone leaves the course with a fully-fledged AI PRD for their portfolio.
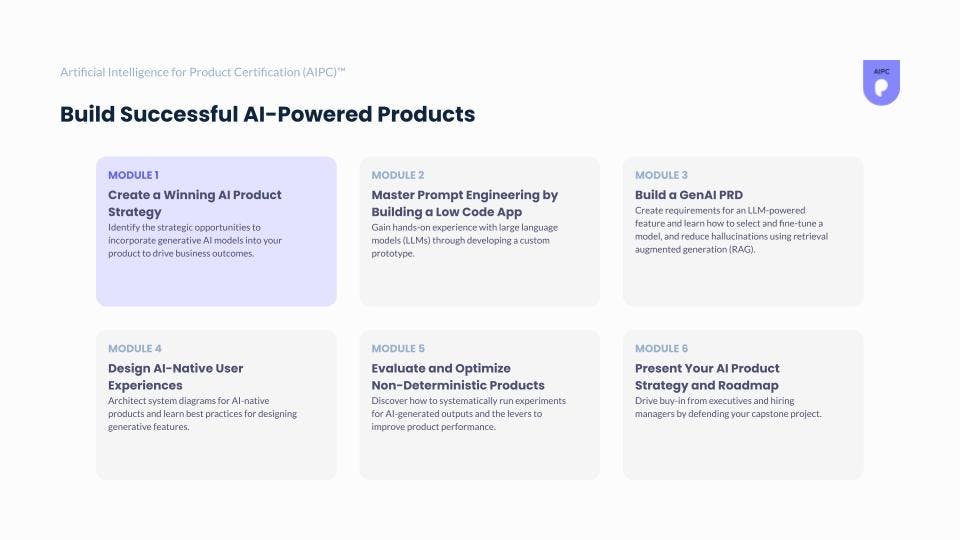
Preparing for an AI/ML PM interview
Ok, so you've applied to AI/ML jobs, the recruiters loved your AI product manager resume, and they offered you the chance to interview. As you set your sights on a role in AI/ML product management, you may wonder how best to prepare for AI product management interview questions. Let’s break down the key areas you should focus on to ace your AI/ML PM interview.
1. Product sense
This involves a deep understanding of the product's core purpose, its target audience, and its position in the market. But it goes beyond just understanding - it's about anticipating. Can you foresee user needs and preferences? How well can you tailor your product's features and functionalities to meet these expectations?
This ability is a cornerstone in demonstrating your proficiency as a product manager, especially in the AI/ML space where user needs can be complex and ever-evolving.
2. Proficiency in statistics
When stepping into AI/ML product management, your acumen in statistics needs to be top-notch. This isn't just a cursory knowledge of basic stats but a deep dive into p-values, confidence intervals, hypothesis testing, and sampling techniques.
Your interview might delve into these areas, assessing your ability to interpret data and make data-driven decisions. The key here is to blend intuition with statistical knowledge, allowing you to navigate through vast data sets and extract meaningful insights.
3. Setting success metrics
In an AI/ML PM career, success metrics take on a new level of importance. You're expected to not only understand but also articulate the impact of a model's output in a real-world production environment.
This is where prioritization frameworks come into play. They help you structure your thinking process, enabling you to solve problems and make decisions effectively.
Mapping user actions to metrics and contextualizing why these metrics are significant is an essential skill. It's about connecting the dots – how user behavior impacts product performance and, in turn, the overall business objectives.
All PMs Will Be AI PMs One Day (Soon)
Many experts believe we are living on the cusp of the next industrial revolution. Just like in the past, new technologies changed how humans lived down to our very anatomy (that’s a callout to fire, the OG technology), AI is going to infiltrate every aspect of our lives. Artificial Intelligence, for product managers, isn’t just about another career choice. It’s about future-proofing your career. It’s about making sure that you’re a part of the next chapter of human history.
Get Certified as an AI Product Manager
To truly set yourself apart, and stay ahead, learn how to build AI products and integrate AI across the entire product lifecycle. The Artificial Intelligence for Product Certification (AIPC)™ provides hands-on instruction to help you learn the skills and knowledge required to build the next generation of products.
enroll nowUpdated: May 6, 2024