Updated: December 5, 2024- 28 min read
When looking for reasons to implement AI solutions in your business, you won’t have a hard time finding them. In fact, if you keep reading, I’ve outlined the top five most important ones below. Here’s a bonus one: mentioning AI has been shown to attract 15% to 50% more investment. However, if you’re reading this article it might be because you’re wary about the hype around AI and wondering if going down this rabbit hole makes sense for your business.
While in the short-term, it’s fair to argue that the 15%-50% increase cited above is not always justified, in the long run, experts agree that this technology will change everything in the same way that the combustible engine and the internet disrupted society and commerce. One day soon, there won’t be AI businesses. There will just be businesses. The ones who didn’t find a way to implement AI in their operations or products will cease to exist.
It’s not just technologists who think this way, either. Business leaders are also starting to see the writing on the wall, with fewer CEOs agreeing they can keep doing what they’re doing. The number of CEOs with faith in business as usual dropped by 6% in just the last year:
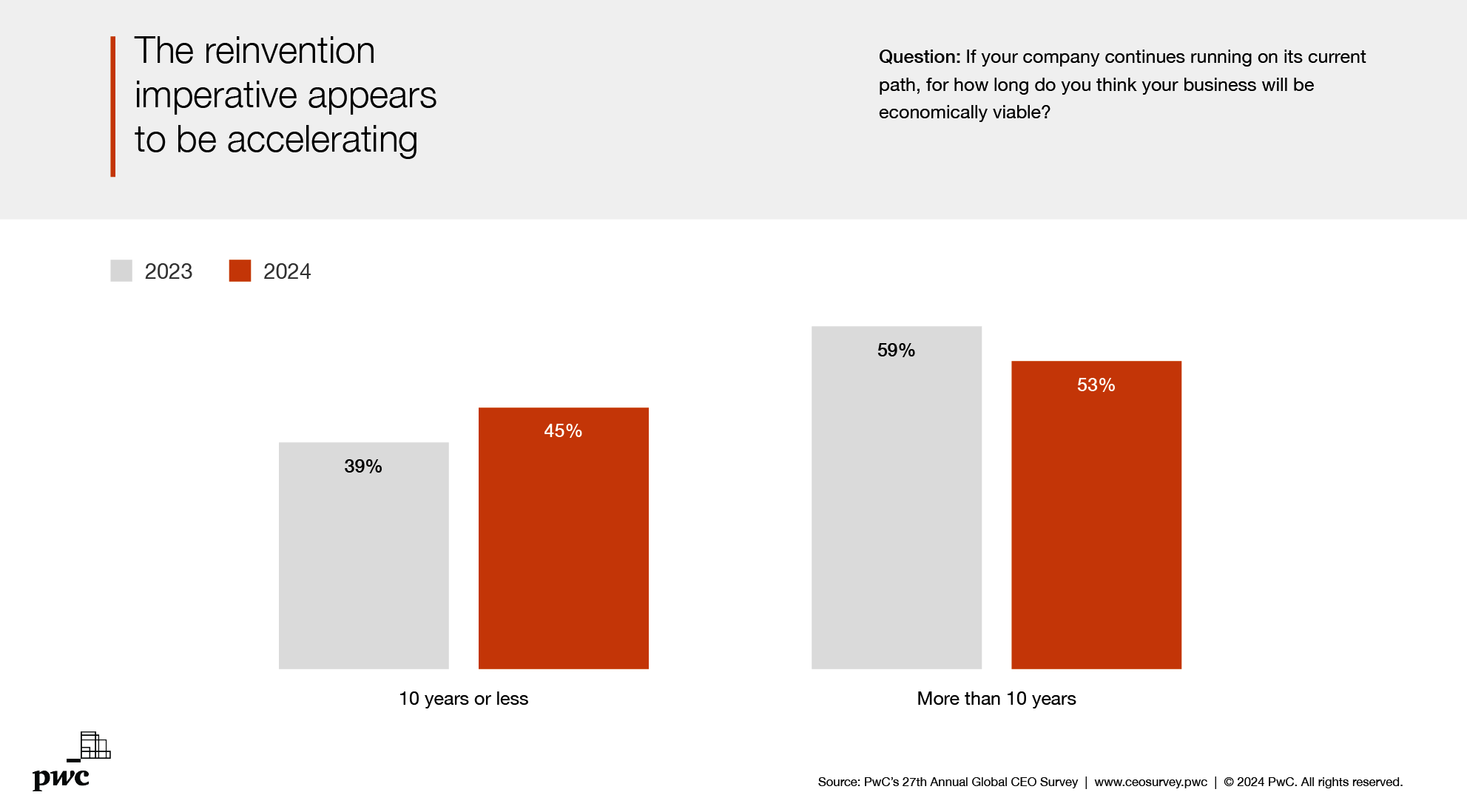
In this article, we’ll cover:
The benefits of AI
Common use cases
Different types of AI
AI implementation strategy:
Roadmap
Prerequisites for success
Common mistakes
Solutions that guarantee AI transformation
Get into the details with AI product development insights from Product School experts with our AI guide:
Level up on your AI knowledge
Take a deep dive into the world of AI with Product School's definitive guide.
Download Guide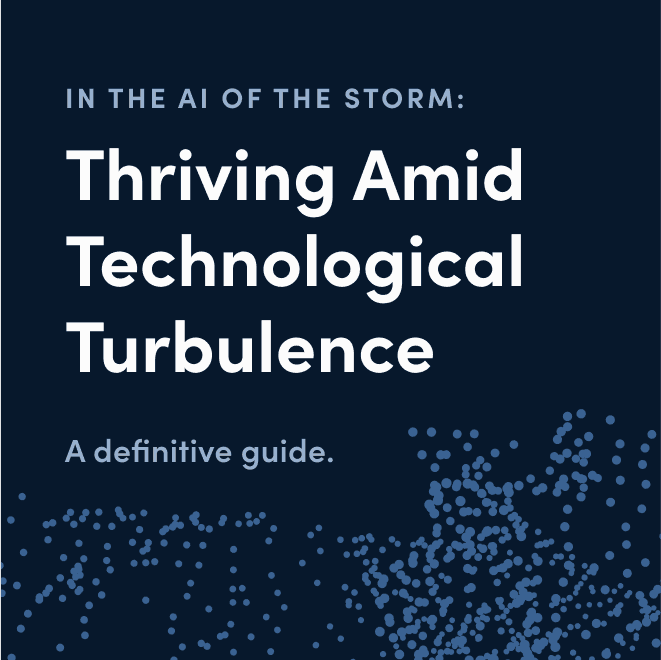
Benefits of Implementing Artificial Intelligence
Implementing artificial intelligence (AI) in business is not just about adopting a new technology; it’s about transforming operations, enhancing customer experiences, and driving significant growth.
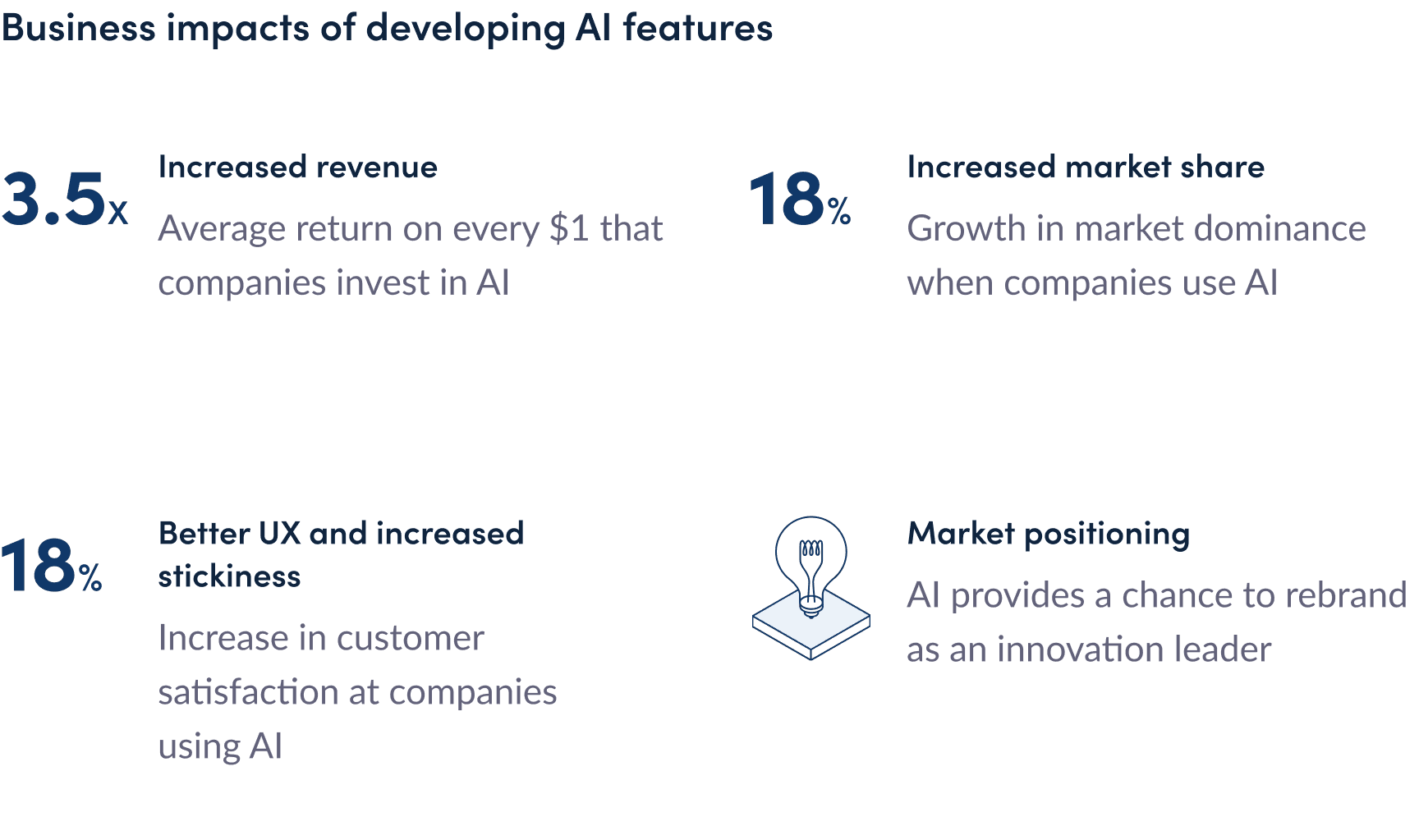
Source: IDC
Boosting Efficiency and Automation
One of the most immediate advantages of AI is its capacity to automate repetitive tasks and optimize business processes. AI technologies like Natural Language Processing (NLP) and machine learning algorithms enable companies to reduce the time and effort required for routine operations. AI can streamline administrative tasks such as data entry and invoice processing, allowing employees to focus on more strategic activities.
Employees can use NLP (Natural Language Processing) to query internal databases and find information on policies and other stored internal knowledge in seconds without having to wait for a response from HR or other departments where information might ordinarily be siloed.
According to research, 24% of use cases driving adoption pertain to processing, sharing, and summarizing documents while a significant portion are related to employee self-service and 22% have to do with process automation in general.
Enhancing Decision-Making with AI
Artificial intelligence significantly improves decision-making by analyzing vast amounts of data to generate actionable insights. Businesses leveraging AI-powered analytics can process complex datasets to uncover patterns and trends that were previously invisible. This capability is crucial for making informed strategic decisions.
AI models provide deeper insights essential for business leaders, including:
Market dynamics
Customer behavior
Operational performance
Personalizing Customer Experiences
AI technologies such as Natural Language Processing (NLP) and machine learning play a crucial role in enhancing customer interactions by delivering personalized experiences at scale. By analyzing customer data, AI systems can provide tailored recommendations, support, and content, which significantly boosts customer satisfaction and loyalty.
AI-powered chatbots and virtual assistants further enhance this personalization by offering immediate, around-the-clock customer support, efficiently resolving queries and improving overall customer engagement. This ability to personalize and streamline customer service interactions is pivotal in today's competitive market.
Driving Innovation and Gaining a Competitive Edge
AI is a catalyst for innovation, providing businesses with the tools to develop new products and services and maintain a competitive edge. Integrating AI into product development and strategic planning opens the door to creating unique value propositions and differentiating offerings in the marketplace.
As researchers at McKinsey point out, AI can “accelerate the selection of proteins and molecules best suited as candidates for new drug formulation.” Experts at Gartner predict that by next year, more than 30% of new drugs will be discovered using AI. The same blend of advanced analytics and machine learning can be applied to other types of product design like electrical circuits.
Cutting Costs and Increasing Profitability
By streamlining operations and optimizing resources, AI can lead to significant cost reductions and improved profitability. The automation of processes and predictive maintenance reduces the costs associated with manual labor and equipment downtime, directly impacting the bottom line.
AI also plays a vital role in resource optimization, such as better managing inventory levels or staffing requirements to minimize waste and maximize output.
“We're seeing that the early adopters who overcame barriers to deploy AI are making further investments, proving to me that they are already experiencing the benefits from AI.”
— Rob Thomas, Senior Vice President, IBM Software
Common Use Cases: How to Implement AI in Your Business
Artificial intelligence (AI) is rapidly becoming an essential tool across various business functions, offering diverse applications that significantly enhance operational efficiency, customer experience, and strategic decision-making. Here are some of the most impactful use cases of AI in business, illustrated by relevant statistics that highlight their effectiveness and widespread adoption.
Customer Service and AI-Powered Support
AI promises productivity gains of 30-45% in the customer service sector via efficient and personalized support through chatbots and virtual assistants. These AI tools are trained on internal documentation and utilize Natural Language Processing (NLP) to interact with customers in real time, offering solutions that range from addressing simple queries to managing complex transactions.
For example, chatbots can handle multiple customer interactions simultaneously, providing 24/7 support without human intervention increasing First Contact Resolution (FCR). When people do intervene, it’s to handle more complicated requests, which they have more time for as AI chatbots handle the simple tasks.
Data Analysis and Predictive Analytics
AI excels at processing and analyzing vast amounts of data swiftly and accurately, making it an indispensable tool for data analysis and predictive analytics in business. Companies leverage AI to gain deeper insights into market trends, customer behaviors, and operational performance, enabling more informed and strategic decision-making.
For instance, AI-driven data analytics tools can help businesses identify emerging market trends and customer preferences, allowing them to adapt their strategies proactively. In operational contexts, AI's predictive capabilities are invaluable; it can forecast demand, predict maintenance needs in manufacturing, and anticipate supply chain disruptions. These predictive insights enable businesses to optimize their operations and reduce costs.
Sales and Marketing Optimization
AI significantly enhances sales and marketing functions by providing sophisticated tools for automating tasks and generating intelligent insights. It is estimated that generative AI could increase productivity in marketing by 5-15%. AI helps marketing and sales teams in the following areas:
Content creation
Lead scoring
SEO and content personalization
Customer data analysis
Supply Chain and Logistics Management
In supply chain and logistics, AI provides advanced capabilities for forecasting demand, managing inventory, and optimizing delivery routes. These improvements help businesses create more efficient, responsive, and resilient supply chains. 18% of 2024 IBM survey respondents reported using AI for supply chain intelligence.
AI can accurately predict product demand based on historical data and market trends, helping companies maintain optimal inventory levels and reduce the risks of overstocking or stockouts. Furthermore, AI-powered logistics solutions analyze various factors such as traffic patterns and weather conditions to optimize delivery routes, reducing transportation costs and improving delivery times.
Fraud Detection and Security
AI plays a crucial role in enhancing security and detecting fraudulent activities by monitoring transactions in real time, identifying suspicious behavior, and alerting businesses to potential threats. AI solutions in finance, where quick and accurate fraud detection can prevent losses, has seen significant gains in the last years.
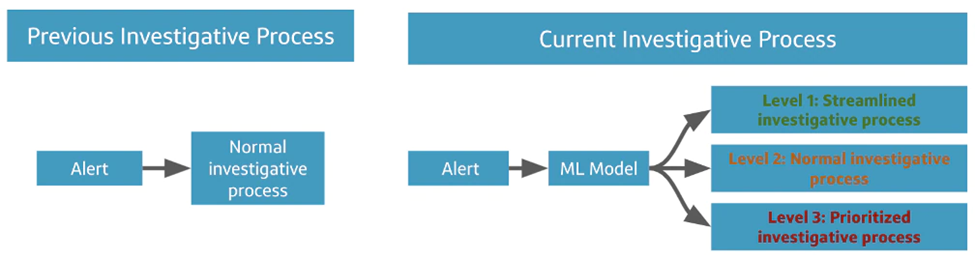
Image courtesy of HBS Digital Initiative
AI systems continuously analyze transaction data and user behaviors to detect anomalies that may indicate fraudulent activities. These systems can also predict and prevent cyber-attacks by recognizing patterns associated with malicious behavior, providing a proactive defense against security threats.
Types of AI Technologies to Incorporate
Implementing artificial intelligence (AI) in business involves various technologies, each with distinct capabilities and applications. Understanding these different types of AI technologies is crucial for selecting the right solutions to address specific business needs. Here’s a look at some of the most significant AI technologies currently transforming industries:
Machine Learning (ML)
Machine Learning (ML) is a subset of AI focused on building systems that can learn from and make decisions based on data. Instead of being explicitly programmed to perform a task, ML algorithms use statistical techniques to identify patterns in data and improve their performance over time.
Supervised Learning: This approach involves training an algorithm on a labeled dataset, where the input-output pairs are known. It's commonly used for tasks like classification (e.g., spam detection in emails) and regression (e.g., predicting house prices).
Unsupervised Learning: In this method, the algorithm is provided with data without explicit instructions on what to do with it. It identifies hidden patterns or intrinsic structures in the data, which is useful for clustering (e.g., customer segmentation) and association tasks (e.g., market basket analysis).
Reinforcement Learning: This involves training algorithms through a system of rewards and penalties. It’s particularly effective in dynamic environments where the algorithm needs to make a series of decisions, such as in robotics and gaming.
Natural Language Processing (NLP)
Natural Language Processing (NLP) enables machines to understand, interpret, and generate human language. NLP is crucial for applications that involve human-computer interaction and data analysis from text.
Language Translation: NLP powers translation services like Google Translate, allowing for real-time communication across different languages.
Sentiment Analysis: This involves analyzing text to determine the sentiment expressed, which is particularly useful for monitoring customer feedback on social media or reviews.
Chatbots and Virtual Assistants: NLP is the backbone of conversational AI systems that can understand and respond to user queries, enhancing customer support and engagement.
Computer Vision
Computer Vision is a field of AI that trains computers to interpret and make decisions based on visual data from the world, such as images and videos. It’s widely used in applications where the system needs to process and analyze visual information.
Image Recognition: This technology can identify objects, people, and other elements within an image. It’s used in various applications, from medical imaging to facial recognition systems.
Video Analysis: AI systems can analyze video content to detect activities, track objects, and even interpret emotions. This is valuable in areas like surveillance and content moderation.
Augmented Reality (AR): Computer vision is integral to AR technologies, which overlay digital information onto the real world, enhancing user experiences in gaming, retail, and more.
Robotic Process Automation (RPA)
Robotic Process Automation (RPA) involves using software robots to automate highly repetitive and routine tasks that are traditionally performed by human workers. RPA is particularly effective in processes that involve interacting with digital systems.
Data Entry and Management: RPA can automate tasks like data extraction, form filling, and data migration between systems, reducing errors and freeing up human resources.
Transaction Processing: In finance, RPA can handle tasks such as processing invoices, handling payroll, and managing account reconciliations.
Customer Service Operations: RPA bots can automate parts of customer service workflows, such as managing queries and processing requests, leading to faster response times and improved customer satisfaction.
Deep Learning
Deep Learning, a subset of machine learning, involves neural networks with many layers (hence "deep") that can model complex patterns in large datasets. It’s particularly powerful in applications where massive amounts of data and intricate pattern recognition are required.
Speech Recognition: Deep learning models are used to transcribe spoken language into text, playing a key role in virtual assistants like Siri and Alexa.
Autonomous Vehicles: These systems rely on deep learning to interpret sensor data and navigate environments safely.
Healthcare Diagnostics: Deep learning algorithms analyze medical images to assist in diagnosing diseases, enhancing the accuracy and speed of medical evaluations.
Developing an AI Implementation Strategy
Implementing artificial intelligence (AI) in your business requires a well-thought-out strategy that aligns with your organization’s goals and capabilities. Developing a robust AI implementation strategy involves several critical steps to ensure successful deployment and integration of AI technologies. Here's a comprehensive guide to crafting an effective AI strategy for your business:
Define Business Objectives for AI Implementation
Before diving into AI technologies, it’s essential to establish clear business objectives. Determine what you aim to achieve with AI—whether it’s improving operational efficiency, enhancing customer experiences, driving innovation, or reducing costs. These objectives will guide your AI initiatives and help measure their success.
Align with Business Goals: Your AI strategy should be closely aligned with your overall business strategy. This alignment ensures that AI projects contribute directly to achieving key business outcomes and priorities.
Set Measurable Goals: Define specific, measurable goals for your AI initiatives. For example, if your objective is to improve customer service, set targets such as reducing response times or increasing customer satisfaction scores.
Assess Current Capabilities and Resources
Evaluate your existing capabilities and resources to understand what you have and what you need for successful AI implementation. This assessment should cover your data infrastructure, technology stack, and human resources.
Data Readiness: AI relies heavily on data. Ensure your organization has access to high-quality, relevant data and robust data management practices. Assess the completeness, accuracy, and accessibility of your data sources.
Technology Infrastructure: Evaluate your current IT infrastructure to determine if it can support AI technologies. Consider factors like computational power, storage capacity, and the availability of advanced analytics tools.
Talent and Skills: Assess your team’s skills and identify gaps. You may need to hire new talent or train existing employees in AI and data science. According to a Gartner survey, 48% of organizations cite a lack of skilled staff as the biggest barrier to AI adoption Gartner.
Identify High-Impact Areas for AI Integration
Focus your AI efforts on areas where they can deliver the most significant impact. Look for processes and operations that are data-intensive, repetitive, or currently hindered by inefficiencies.
Process Automation: Identify tasks that can be automated to free up human resources and reduce operational costs. Robotic Process Automation (RPA) is an example of AI that automates routine, rule-based tasks.
Customer Interaction: Consider implementing AI in customer-facing roles, such as using chatbots for customer service or personalized marketing recommendations to enhance customer engagement.
Operational Efficiency: Use AI to optimize logistics, supply chain management, or predictive maintenance in manufacturing, where AI can predict failures and schedule maintenance before issues arise.
Create a Roadmap for AI Implementation
Develop a detailed roadmap that outlines the steps for implementing AI in your business. This roadmap should include timelines, key milestones, and resource allocation.
Start with Pilot Projects: Begin with small-scale pilot projects to test AI applications in a controlled environment. These pilots help validate the feasibility and effectiveness of AI solutions before scaling up.
Set Realistic Timelines: Establish realistic timelines for each phase of the AI implementation. Consider potential challenges and allocate time for testing, training, and refinement.
Allocate Resources: Ensure that adequate resources—financial, technological, and human—are allocated to support the AI initiatives. This includes budgeting for technology investments and personnel training.
Build or Source AI Talent
AI implementation requires a skilled workforce that understands both the technical and business aspects of AI. Building or sourcing the right talent is crucial for success. 42% of companies cite lack of talent is cited as one of the top barriers to AI adoption.
In-House Training: Train your existing staff in AI technologies and methodologies. Investing in upskilling can be more cost-effective and beneficial as your team already understands your business context.
Hiring Experts: If the required skills are not available internally, consider hiring data scientists, AI specialists, or partnering with AI consulting firms.
AI Training for Teams
Accelerate revenue and 2x productivity when you equip teams across your organization with AI skills and training.
Learn MoreSelect the Right AI Tools and Technologies
Choosing the right AI tools for your business is critical. Evaluate solutions that best fit your business needs and technical environment.
Platform and Tools: Select AI platforms and tools that are scalable and integrate well with your existing systems. Consider factors like ease of use, support, and compatibility with your current technology stack.
Vendor Partnerships: Partner with technology vendors that offer robust AI solutions and support services. These partnerships can provide access to advanced technologies and expertise that may not be available in-house.
Selecting and Training a Large Language Model (LLM)
If building a product powered by Gen AI or designing a large-scale project powered by NLP (Natural Language Processing) you will need to consider integrating a Large Language Model (LLM) into your AI strategy. This requires careful consideration and planning to ensure it meets your specific needs and performs effectively.
Choose the Right LLM for Your Use Case: Consider factors like model size, computational requirements, and pre-trained knowledge to align with your project goals.
Customize and Fine-Tune the Model: Fine-tune the selected LLM on domain-specific data to enhance its relevance and accuracy for your particular application. Utilize transfer learning to adapt the model to your needs without starting from scratch, saving time and resources.
Establish Training and Evaluation Protocols: Use diverse datasets for training and validation to ensure the model generalizes well and performs reliably in various scenarios. Implement regular performance evaluation and testing against real-world scenarios to refine and optimize the model's accuracy and efficiency.
Learn more about LLMs and how to optimize them in Product School’s AI guide:
Level up on your AI knowledge
Take a deep dive into the world of AI with Product School's definitive guide.
Download Guide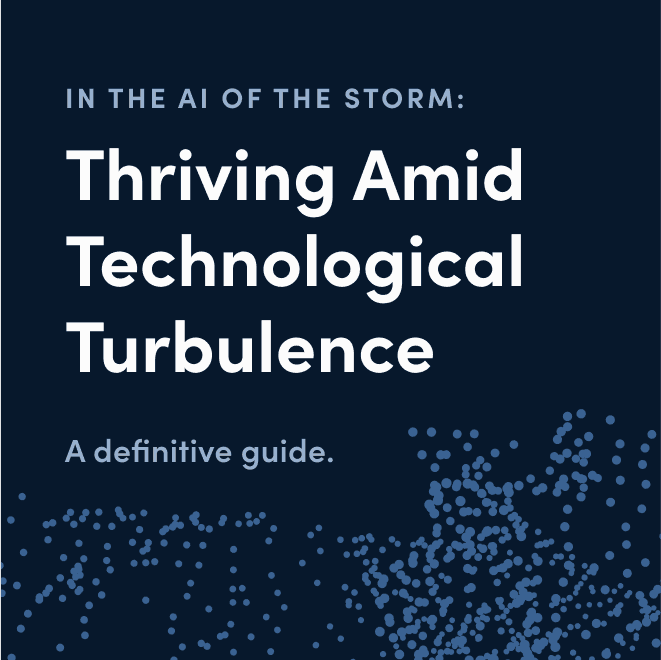
Pilot AI Projects and Scale Up
Start with small, manageable AI projects to pilot the technologies and strategies in real-world conditions. These pilot projects serve as a test bed for refining your approach and demonstrating value.
Evaluate and Learn: Assess the outcomes of pilot projects against your objectives. Use these insights to understand what works, identify challenges, and adjust your strategy as needed.
Scale Successful Initiatives: Once a pilot project proves successful, develop a plan to scale the solution across the organization. Ensure that scaling is supported by adequate resources and infrastructure.
Monitor and Iterate Your AI Strategy
Continuous monitoring and iteration are vital to keep your AI initiatives aligned with evolving business needs and technological advancements.
Performance Metrics: Regularly measure the performance of AI systems against your set objectives and key performance indicators (KPIs). Use these metrics to gauge success and identify areas for improvement.
Adapt and Evolve: AI technologies and business environments are constantly changing. Stay agile and be ready to adapt your AI strategy to new challenges and opportunities. Learning from each implementation phase will enhance your approach and ensure sustained value creation.
Prerequisites for Successful Implementation of Artificial Intelligence
Successful implementation of artificial intelligence (AI) in a business environment requires more than just the adoption of technology. It involves preparing the organization comprehensively to ensure that AI initiatives are effectively integrated and yield the desired outcomes. Here are the key prerequisites for successful AI implementation:
1. Data Quality and Readiness
Data is the lifeblood of AI. The success of AI models heavily depends on the quality, volume, and relevance of the data they are trained on. Before embarking on AI projects, businesses must ensure that their data is clean, accessible, and representative of the issues they intend to address.
High-Quality Data with Accurate Labeling: For AI to be effective, it requires high-quality datasets that are not only clean and well-organized but also accurately labeled. Meticulous annotation and classification of data points provides the necessary context and categorization that enable AI algorithms to learn and make accurate predictions.
Data Accessibility: Data should be easily accessible and organized in a manner that supports AI applications. Implementing a centralized data repository can facilitate easier access and integration of data from various sources.
Data Security and Compliance: With the increasing focus on data privacy regulations like GDPR and CCPA, it’s crucial to ensure that your data practices comply with legal requirements. Secure handling of data is essential to avoid breaches and maintain customer trust.
2. Robust Technology Infrastructure
A robust and scalable technology infrastructure is essential for supporting the computational demands of AI applications. This includes both hardware and software capabilities necessary to process large datasets and run complex algorithms.
Computational Resources: AI applications, particularly those involving deep learning, require significant computational power. Investing in high-performance computing (HPC) resources or leveraging cloud computing platforms can provide the necessary scalability and processing power.
Integration Capabilities: Your technology stack should be capable of integrating AI tools and platforms seamlessly. This includes compatibility with existing systems and the ability to support various AI technologies and frameworks.
3. Skilled Workforce for AI
Implementing AI requires a diverse set of skills, from data science and machine learning expertise to knowledge in specific business domains. Building or sourcing a skilled workforce is crucial for the success of AI projects.
AI Specialists: Data scientists, machine learning engineers, and AI researchers are essential for developing and deploying AI models. These roles require strong analytical skills and a deep understanding of AI technologies.
Domain Experts: In addition to technical expertise, it’s important to have domain experts who understand the business processes and challenges that AI aims to address. They play a key role in guiding the application of AI to real-world problems and ensuring alignment with business goals.
Cross-Functional Collaboration: Encourage collaboration between data scientists, IT professionals, and business leaders. This cross-functional approach ensures that AI initiatives are well-integrated and supported across different parts of the organization.
4. Executive Leadership and Support
Strong leadership and support from the top levels of the organization are critical for driving AI adoption and overcoming resistance to change. Executive buy-in ensures that AI projects receive the necessary resources and strategic alignment.
Vision and Commitment: Leaders must articulate a clear vision for how AI will benefit the organization and commit to supporting AI initiatives through investment and resource allocation Deloitte.
Change Management: Effective change management strategies are essential for addressing cultural and operational shifts that accompany AI implementation. This includes preparing employees for new roles and processes and fosters an environment that embraces innovation.
5. Effective Change Management
Introducing AI into an organization often requires significant changes in workflows, roles, and company culture. Effective change management is necessary to ensure that these changes are smoothly integrated and embraced by employees.
Training and Upskilling: Provide training programs to help employees understand AI technologies and how they will be used in their roles. This can reduce resistance and increase acceptance and proficiency with AI tools.
Communicating Benefits: Clearly communicate the benefits of AI to the organization and how it will improve business outcomes.
Supporting Adaptation: Provide ongoing support as employees adapt to new AI-driven processes. This might include access to resources, continuous learning opportunities, and a platform for feedback and improvements.
Common Mistakes in AI Implementations and How to Avoid Them
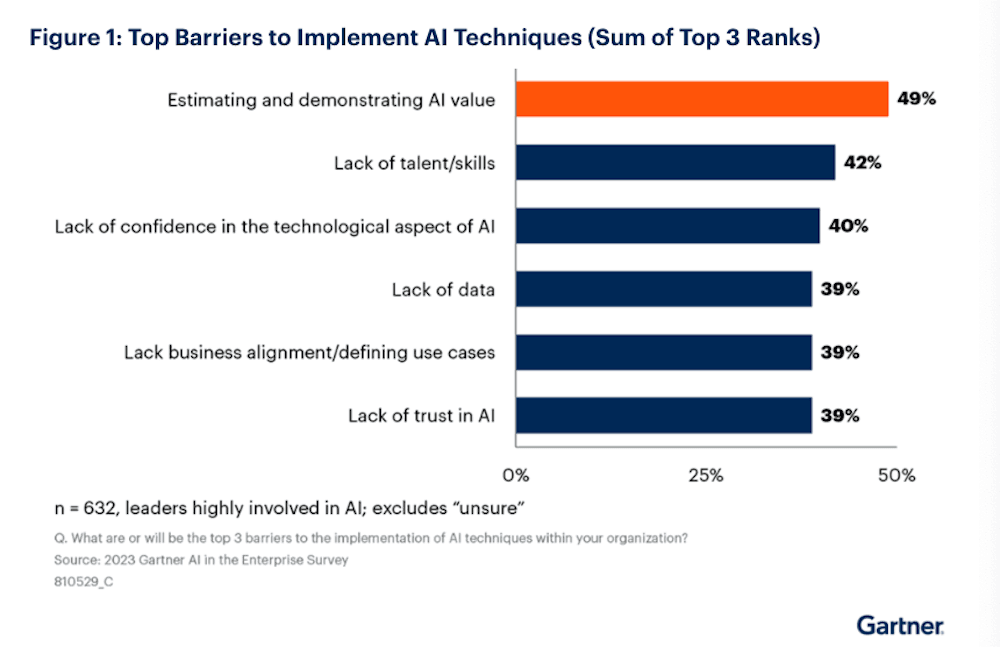
Source: Gartner (May 2024)
Implementing artificial intelligence (AI) in a business setting offers significant potential benefits, but it also presents a set of challenges. Many organizations encounter pitfalls that can hinder their AI initiatives. Here are some common mistakes in AI implementations and how to avoid them.
Lack of Clear Objectives for AI Implementation
One of the most fundamental mistakes businesses make is embarking on AI projects without a well-defined purpose. Implementing AI technology without clear goals often leads to wasted resources and unfulfilled expectations.
Undefined Goals: Without specific objectives, it’s challenging to measure the success of AI initiatives. Organizations might deploy AI for the sake of having the technology without understanding what they want to achieve, such as improving customer service, reducing costs, or increasing sales.
Misalignment with Business Strategy: AI projects that are not aligned with the overall business strategy can struggle to demonstrate value. AI should be used as a tool to achieve strategic business goals, not as an end in itself.
Solution: Define clear, measurable goals that align with your business strategy. Ensure that every AI initiative is driven by a specific business need or opportunity and has defined success metrics.
Inadequate Data Management
AI systems are only as good as the data they are trained on. Poor data quality, fragmented data sources, and lack of data governance can severely impair the effectiveness of AI solutions. Research shows that too much data complexity holds back 1 in 4 companies from enjoying the full benefits of AI.
Poor Data Quality: Inaccurate, incomplete, or outdated data can lead to erroneous AI predictions and insights. Quality issues in data can undermine the reliability and effectiveness of AI applications.
Fragmented Data Sources: Data silos within organizations can prevent AI systems from accessing the comprehensive data they need. AI thrives on integrated datasets that provide a holistic view.
Lack of Data Governance: Without proper data governance, managing data privacy, security, and compliance becomes challenging, especially with increasing regulatory scrutiny.
Inconsistent or Incomplete Data Labeling: Data labeling is a critical step in preparing datasets for AI training, especially in supervised learning tasks where labeled examples guide the model’s learning process. Inconsistent or incomplete labeling can significantly degrade the performance of AI models by introducing noise and ambiguity.
Solution: Invest in data quality management and integration efforts. Establish strong data governance practices to ensure data is accurate, accessible, and secure. A robust data strategy is foundational for successful AI implementation.
Underestimating Costs and Timelines
AI projects often require significant investment in technology, infrastructure, and talent. Underestimating the costs and timelines associated with AI initiatives can lead to budget overruns and project delays.
Cost Underestimation: Many organizations overlook the full spectrum of costs involved in AI implementation, including hardware, software, training, and ongoing maintenance.
Timeline Misjudgment: AI projects can take longer to develop and deploy than initially anticipated, particularly when dealing with complex systems and integration issues. Rushed implementations can result in suboptimal performance and missed objectives.
Solution: Develop a realistic budget and timeline for AI projects, considering all potential costs and stages of implementation. Allow time for testing, iteration, and refinement to ensure robust and reliable AI solutions.
Ignoring Ethical and Regulatory Considerations
Ethical and regulatory issues surrounding AI are increasingly important as these technologies become more pervasive. Failing to address these considerations can lead to legal challenges, reputational damage, and loss of customer trust.
Data Privacy and Security: AI systems that process personal data must comply with data protection regulations like GDPR and CCPA. Mishandling sensitive information can result in severe penalties and loss of consumer confidence.
Bias and Fairness: AI algorithms can inadvertently perpetuate biases present in the training data, leading to unfair outcomes. It’s crucial to ensure AI systems are designed and tested to avoid discriminatory practices.
Transparency and Accountability: Stakeholders often require clarity on how AI decisions are made. Lack of transparency can create trust issues, especially in critical applications like finance or healthcare.
Solution: Incorporate ethical guidelines and regulatory compliance into your AI strategy. Conduct regular audits to ensure AI systems operate fairly and transparently. Develop clear policies for handling data and addressing potential biases.
Challenges in Integrating AI with Existing Systems
Integrating AI solutions into existing business processes and systems can be complex. Many organizations face difficulties in embedding AI into their current workflows and infrastructure.
Compatibility Issues: AI tools may not seamlessly integrate with legacy systems, leading to data silos and operational inefficiencies. This disconnect can limit the effectiveness of AI solutions.
Operational Disruption: Introducing AI can disrupt established workflows, causing temporary declines in productivity and employee resistance if not managed properly.
Solution: Plan for integration from the outset, ensuring that AI solutions are compatible with existing systems. Use pilot projects to test integrations and refine approaches before full-scale deployment. Engage IT and operations teams early to facilitate smooth integration.
Resistance to Change in AI Adoption
AI implementation often requires significant changes in how work is performed and decisions are made. Organizational resistance to these changes can impede the successful adoption of AI.
Cultural Barriers: Employees may fear job displacement or struggle to adapt to new AI-driven processes. A lack of understanding and trust in AI can lead to resistance and reduced engagement.
Change Management Gaps: Failing to effectively manage the transition to AI can result in low adoption rates and underutilization of AI capabilities.
Product School's AI Implementation Solutions
Implementing artificial intelligence (AI) in your business can be a complex and challenging process. It requires not only the right technology and data but also skilled professionals who understand both AI and your business context. Product School offers a range of services and training programs designed to help businesses navigate the intricacies of AI implementation and maximize their potential. Here’s how Product School can support your AI journey:
Supporting Your AI Transformation
Whether you are just beginning your AI journey or looking to scale your existing AI capabilities, Product School can provide the support and expertise you need to succeed.
Strategy and Planning: Product School can help you develop a comprehensive AI implementation strategy that aligns with your business goals. From assessing your current capabilities to identifying high-impact areas for AI deployment, they guide you through each step of the planning process.
Implementation Support: During the implementation phase, Product School’s experts can assist with integrating AI technologies into your existing systems and processes. They offer practical advice on overcoming common challenges and ensuring a smooth transition.
Continuous Improvement: After the initial implementation, Product School continues to support your AI initiatives through ongoing training and mentorship. This ensures that your team can adapt to new developments in AI and continue to optimize their use of AI technologies.
Comprehensive AI Training Programs
AI Training for Teams
Accelerate revenue and 2x productivity when you equip teams across your organization with AI skills and training.
Learn MoreAccording to a recent IBM survey, only 34% of companies are reskilling employees in AI. This presents an enormous opportunity for those companies who reap more benefits from AI investments and increase employee retention and engagement.
Product School provides training programs focused on AI and product management that can equip your team with the necessary skills and knowledge to implement AI effectively.
AI for Product Managers: This program is tailored for product managers who need to understand AI concepts and how they can be applied to develop innovative products. It covers key AI technologies, how to integrate AI into product development, and how to manage AI projects effectively.
Executive Advisory: For senior leaders and executives, Product School offers coaching and advisory services that focus on strategic aspects of AI implementation to help leaders leverage AI to drive business growth and make informed decisions.
Tailored Corporate Training Solutions
Every business has unique needs and challenges when it comes to AI implementation. Product School offers customized training solutions that are tailored to the specific requirements of your organization.
Customized Curriculum: Product School works with businesses to develop training programs that are aligned with their strategic goals and industry-specific needs. Whether you need to upskill your entire team or focus on specific roles, Product School can design a curriculum that fits your objectives.
On-Site and Virtual Training: Flexible training delivery options ensure that your team can learn in a way that suits them best. Product School offers both on-site training sessions and virtual classes, allowing for a seamless integration of learning into your employees’ schedules.
Continuous Learning and Support: Beyond initial training, Product School provides ongoing support and resources to help your team stay up-to-date with the latest advancements in AI. This continuous learning approach ensures that your organization remains competitive and agile in a rapidly evolving landscape.
Access to a Network of Industry Experts
Product School connects your business with a vast network of AI and product management experts who can provide valuable insights and guidance.
Expert Instructors: Product School’s courses are taught by seasoned professionals who have extensive experience in AI and product management. They bring real-world knowledge and industry best practices to the classroom, offering practical advice that goes beyond theoretical learning.
Coaching and Advisory: Beyond classroom instruction, Product School offers mentorship and coaching services. This personalized support helps your team navigate the challenges of AI implementation and apply their learning effectively in their roles.
Community and Networking: Joining Product School’s programs also means becoming part of a global community of product managers, data scientists, and AI professionals. This network provides opportunities for collaboration, knowledge sharing, and professional growth.
By partnering with Product School, your business gains a trusted advisor and partner in your AI transformation journey, helping you harness the full potential of AI to drive innovation and growth.
AI Implementation Strategy: Impact and Effort
Crafting a successful AI implementation strategy requires balancing the potential impact and the effort involved. From setting clear objectives and preparing high-quality, labeled data to selecting and fine-tuning appropriate technologies like Large Language Models, each step demands careful planning and execution. The impact of AI is maximized when data quality and model relevance are prioritized, ensuring reliable and efficient outcomes. Effort is well-spent on fostering AI literacy and overcoming the skills gap barrier. Lighter lifts might include using generative AI for content and coding.
The important thing is that your business needs to start yesterday. Whether by tackling quick wins or preparing for big bets, there’s no time to waste because competitors are already increasing productivity and market share with AI. That said, there’s no need to panic. By staying adaptable and informed, businesses can leverage AI to drive significant innovation and sustainable growth, turning strategic efforts into impactful results.
Future proof your team and discover how we can help you build Product excellence in your organization
Schedule a callUpdated: December 5, 2024