Updated: October 28, 2024- 13 min read
One of the most salient applications of artificial intelligence in any business strategy is leveraging the power of machine learning for data analysis. AI models can see patterns that people are incapable of perceiving on their own. In the case of predictive analytics, it can even see into the future. Contemplating AI data analytics, one starts to feel a bit like Little Red Riding Hood: “My, what big eyes it has!”
AI also has big teeth when it comes to analyzing data, and it should come as no surprise that it carries with it risks alongside the benefits. We’ll cover those here, along with a list of six top AI data analysis tools and how companies should use them.
To really explore this topic from a product perspective, I consulted Ash Sharma, an Amazon AI Product Leader who we are lucky enough to have as an instructor at Product School training the next generation of Product Managers in AI. I have no doubt you’ll find his thoughts throughout the article extremely relevant.

Ash Sharma
AI Product Leader at Amazon
Ash Sharma is a visionary product leader with a proven track record in building innovative products and high-performing teams. Currently, he leads Alexa's advancement as a conversational Generative AI assistant at Amazon, where he has made Alexa more helpful, personalized, and accessible.
What Is AI Data Analytics?
Artificial intelligence data analysis is the application of AI technologies to extract insights, detect patterns, and make data-driven decisions from large-scale datasets. This can include anything from machine learning algorithms that predict future trends to natural language processing (NLP) models that enable better interaction with data.
Traditional data analysis involves manual exploration of data, looking for correlations, and summarizing key insights. However, AI-driven data analytics takes this a step further, leveraging machine learning models that can handle huge volumes of data, spot hidden patterns, and learn from new data inputs. With AI analytics, businesses can go beyond conventional methods to unlock value that would otherwise remain hidden.
Machine learning, one of the core techniques used in AI data analysis, allows models to learn from historical data, identify significant trends, and anticipate future outcomes. For example, in customer behavior analysis, AI can determine the likelihood of a customer churning based on past activities.
What’s more, NLP makes it possible to “chat with the data.” In practice, this means asking questions about trends or data points and receiving answers from AI-powered software in natural language. This evolution has made it easier for non-technical team members to interact with data, making companies more data-driven as a whole.
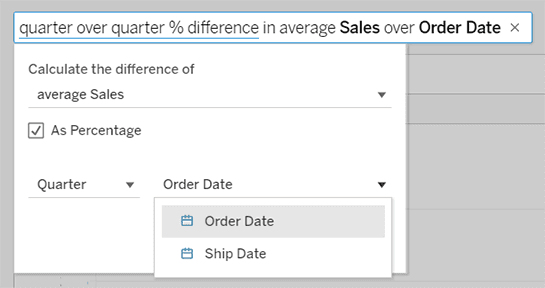
Source: Tableau
AI analytics tools are transforming how businesses operate by streamlining processes that were traditionally labor-intensive and complex. With these tools, data analysis has become faster, more accurate, and scalable, providing insights that are critical for strategic decision-making. As Ash Sharma put it:
“AI can help make sense of the large volume of analytics that are produced and never get in front of stakeholders. Natural language and conversational interfaces are a great value add for non-technical audiences to query an AI system—"which customer segment had the largest repeat usage last week?"—to quickly get insights and disseminate them throughout the organization.”
Applications of AI in Business Analytics
The real power of AI in business analytics lies in its practical applications. Below are some prominent examples of how businesses are using AI for data analytics to gain a competitive edge:
Improving User Experiences with AI Analytics
One of the significant applications of AI analysis is enhancing user experiences. In SaaS, Product teams often rely on session replays to glean insights into how users interact with products and where there might be friction. AI does the same thing but on a whole new scale, leveraging additional touchpoints and types of user behavior that don’t come through in replays.
Sharma explained how this methodology is used in an Escheresque fashion to improve AI-powered products like voice assistants:
“AI assistants have to contend with a large surface area of user queries, tasks, and information requests. If the response or interaction with the assistant is subpar, the user often has to repeat their ask or may respond with frustration. ”
He continued:
“The challenge for product teams is often the inability to accurately detect these user experience issues or understand which particular areas to prioritize. This is where AI models can help—finding patterns among user-perceived defects, e.g. a raised voice, repeating the same ask more emphatically, etc. When a pattern is matched against trillions of data points, statistically acceptable accuracy can be developed in AI models (e.g. classifiers) that can reduce such user-perceived defects. Here, AI-driven analytics help turn a problem of massive scale into something more wieldy to tackle. This methodology is used quite a bit in the industry.”
By identifying these patterns and matching them against trillions of data points, AI models can effectively address user issues and significantly improve overall user experience. AI analytics tools can help turn a massive, seemingly unmanageable problem—like understanding a broad range of user interactions—into a comprehensible range of data points.
Customer Segmentation
Another key use case of using AI for data analysis is in customer segmentation. Businesses can use AI data analysts to create more precise product personas based on user behavior, purchase history, and even real-time data. This segmentation allows for more personalized marketing, ensuring customers receive offers that are relevant to their preferences.
For example, an e-commerce company might use AI-powered analytics to analyze customer purchasing habits and segment customers based on how frequently they purchase, their average spending, and the types of products they prefer. This segmentation enables businesses to tailor their marketing campaigns more effectively, improving engagement rates and driving higher sales.
Sales Forecasting with AI-Powered Analytics
AI analytics also plays a crucial role in forecasting future sales. Traditional sales forecasting often relies on historical sales data and human intuition, which can be prone to error. With AI-driven models, businesses can analyze historical data, seasonality trends, and various external factors to make more accurate sales predictions.
For instance, a retail business can use AI tools for data analysis to forecast product demand by considering not just historical sales but also other variables such as weather conditions, upcoming holidays, and consumer sentiment. The result is a more reliable prediction that helps with inventory management, marketing, and overall business planning.
AI Training for Teams
Accelerate revenue and 2x productivity when you equip teams across your organization with AI skills and training.
Learn MoreThe 6 Best AI Tools for Data Analysts
When it comes to using AI tools for business analytics and predictive intelligence, the variety of options available can be overwhelming. To help you make the right choice, we’ve put together a selection of some of the best AI tools for data analysts based on the capabilities they offer and the business needs they address. These tools range from platforms that integrate AI with traditional analytics to solutions designed to simplify data collaboration and visualization.
1. Mode
Mode is a collaborative data analytics platform that combines SQL, Python, R, and visualization tools to provide a comprehensive analytics experience. With integrated AI analytics, Mode helps data teams find insights faster and share them across the organization. It also provides tools for collaboration, making it easier for data analysts, business stakeholders, and engineers to work together on data-driven projects.
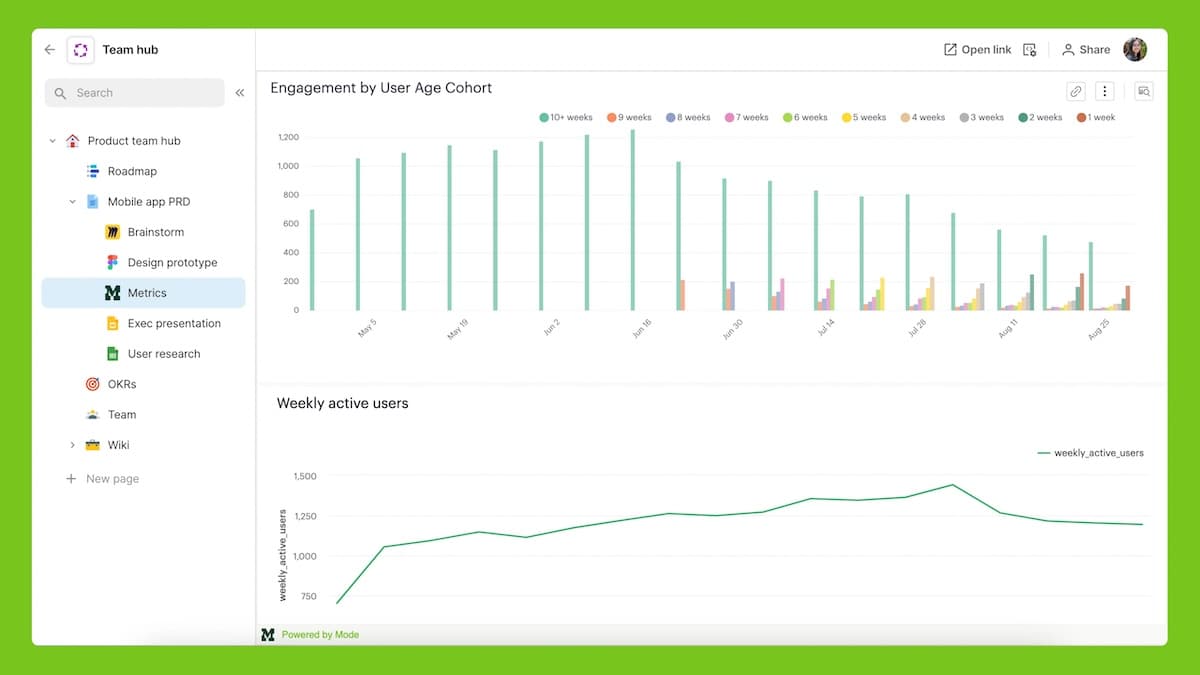
2. Secoda
Secoda is an AI-powered data discovery and collaboration tool. It helps data teams quickly locate data assets, understand context, and improve the overall efficiency of AI data analysts. What’s more, Secoda’s intuitive interface means that even non-technical stakeholders can discover relevant data insights.
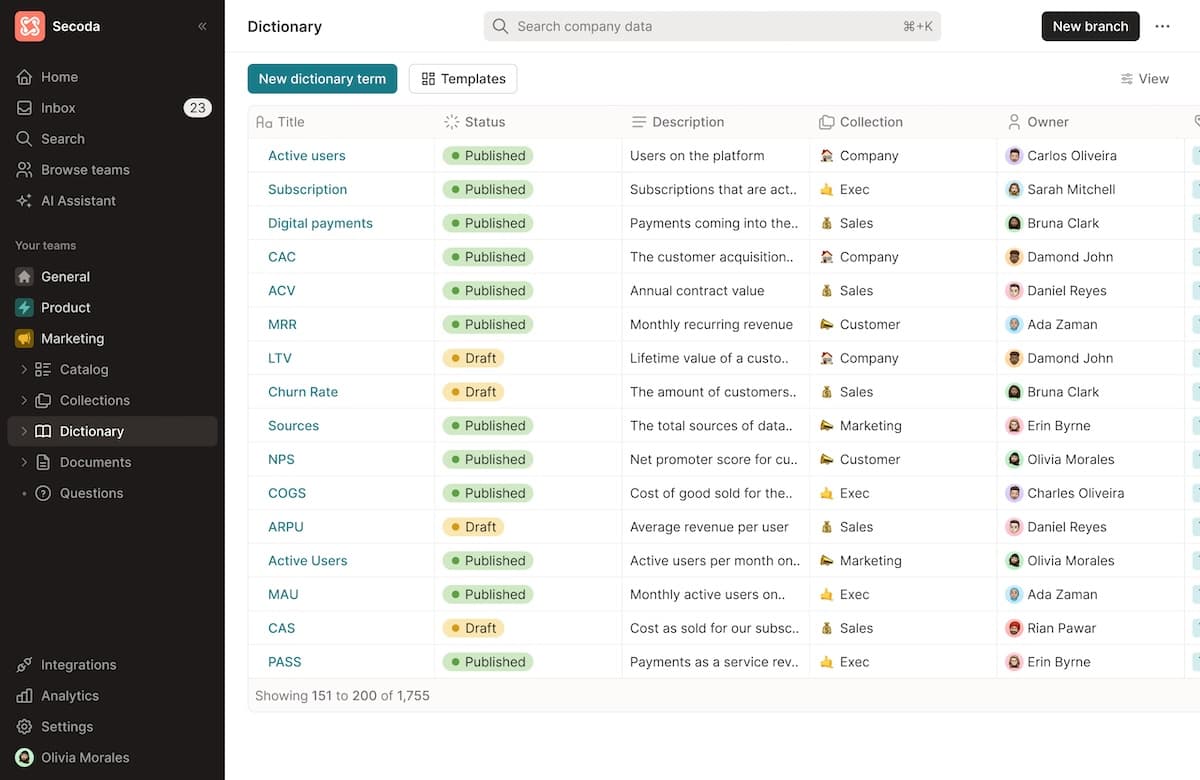
3. Artifact
Artifact is an AI-driven tool that focuses on helping organizations generate actionable business insights. It leverages AI data analysis to automatically generate reports and narratives, offering easy-to-digest summaries that save time for business leaders. The platform also integrates with popular business tools, making it easy to bring AI-generated insights directly into the workflow and bridge the gap between raw data and clear, actionable insights.
4. Google Cloud AI
Google Cloud AI offers comprehensive AI-powered analytics capabilities. With tools for machine learning and big data analytics, Google Cloud AI makes it possible to develop, train, and deploy AI models at scale. Its machine learning capabilities are particularly useful for predictive analytics, allowing data analysts to create models that forecast future outcomes based on historical data.
5. Azure Machine Learning
Azure Machine Learning is another top AI tool that integrates seamlessly with existing Microsoft infrastructure, making it ideal for organizations already using Azure services. It offers pre-built algorithms that simplify the process of building, training, and deploying machine learning models. The tool also includes features like drag-and-drop model building, making it accessible for AI data analysts who may not have a deep programming background.
6. Tableau with AI Integration
Tableau is a popular data visualization tool, but with its AI capabilities (like "Ask Data"), it becomes a powerful tool for using AI to analyze data. It allows users to ask natural language questions, making insights easily accessible to non-technical stakeholders. Tableau’s AI-powered insights can help visualize patterns, anomalies, and trends within datasets, making it easier for businesses to understand and act on their data.
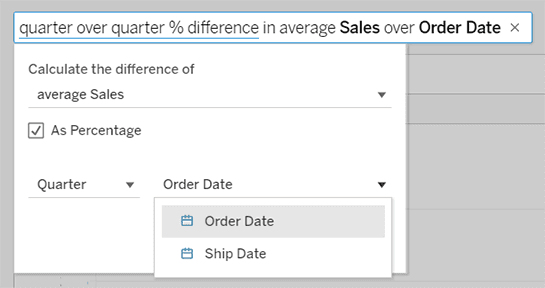
Step-by-Step Tutorial: How to Use AI Tools for Data Analysis
To give you a hands-on sense of how to harness AI data analysis tools, let’s walk through a simple step-by-step guide using Google Cloud AI.
Setup Google Cloud AI
Start by setting up your Google Cloud account and enable Google BigQuery to connect your datasets and perform advanced data analytics.
Data Preparation
Begin by importing the dataset into Google BigQuery.
Google Cloud provides data preparation features to clean up the data by removing inconsistencies, filling in missing values, and transforming data into the correct format for analysis.
Running the AI Analysis
Once the data is prepared, you can use Google AutoML to build your machine-learning model. Choose a model type—such as classification or regression—depending on the business problem you are trying to solve.
For instance, you could use the model to predict customer churn by training it on historical customer behavior data.
Benefits of AI in Data Analytics and Common Mistakes
Benefit #1: Enhanced Insight Generation with AI Data Analysis
One of the biggest benefits of AI in data analytics is its capability to process vast datasets, identify trends, and generate meaningful insights much more quickly and accurately than traditional data analysis methods. AI tools can examine thousands or even millions of data points to discover patterns that humans are incapable of or simply lack the time to process.
Of course, insights are only as useful when they’re applied. Sharma reminded me of this same point when he talked about leveraging the full analytics cycle.
“The largest mistake [businesses make when implementing AI data analytics strategies] is not understanding the full cycle of analytics: Data → Insights → Communication → Comprehension → Corrective Action. Most strategies end at the Insights stage and perhaps a throw-over-the-wall communication. The point of analysis is to make sure the target stakeholders understand it, benefit from it, and will be able to action it towards improvements. ”
If an insight falls in the forest where no one hears it, does it really make a sound? You can have best-in-class tools and excellent AI data analysts generating brilliant insights, but if those insights aren’t communicated, they won’t be impactful.
Benefit #2: Improving Efficiency with AI-Powered Analytics
Another benefit of AI-driven data analytics is its efficiency in improving user experiences. By using AI analytics to detect subtle signals, they can address pain points in a much smoother and more enjoyable experience for the end user.
This type of efficiency also translates to saved costs for the business, as fewer resources are needed for customer support and problem resolution.
The flip side of these efficiency boosts is that it’s easy to take them too far and overcomplicate problems that could be easy fixes. As Sharma stated:
“ 'Just add AI' approaches may sound promising for internal marketing purposes but are often more complex than what is needed. Sometimes, no Gen AI solution is needed, and simple predictive analytics—in use for over 15 years in the industry—is what's called for.”
His advice?
“Be pragmatic; identify real problems, confirm they are urgent and that the solution is actually palatable.”
Benefit #3: Automated Decision Support for Non-Technical Stakeholders
One major hurdle in traditional data analytics is making insights accessible to stakeholders who may not have a strong technical background. AI analytics tools bridge this gap by providing an interface that allows non-technical business leaders to interact directly with data. This might be through dashboards with natural language interfaces or AI-generated insights that are easy to understand and actionable.
“Generative AI can also be used to produce stakeholder presentations, short-form videos, and custom avatars to explain and sell key insights to internal customers in an engaging manner.”
Risks of Using Artificial Intelligence in Data Analytics
Data Privacy in AI Data Analysis
The use of AI for data analytics comes with its own set of risks, and data privacy is one of the most important concerns. AI models often require large amounts of data to generate accurate insights, which can include sensitive information such as customer locations, contact details, and behavioral data. Mishandling this data can lead to significant privacy breaches.
“Personally identifiable data (e.g., locations, phone numbers, etc.) need to be identified by AI precursor systems so that data is properly sanitized. This is particularly important for anything that requires human reviews or annotations to evaluate the AI Assistant's response.”
Data sanitization ensures that no sensitive information is accessible during model training or human reviews, reducing the risk of exposure. Business leaders must prioritize privacy by ensuring AI systems are compliant with regulations like GDPR or CCPA and using techniques such as anonymization and encryption.
Algorithmic Bias in AI Analytics
Another key challenge in using AI for data analysis is addressing algorithmic bias. AI models are only as good as the data used to train them. If the training data is biased, the resulting model will inherit those biases, leading to unfair or incorrect predictions.
Ashish Sharma emphasized:
“Algorithmic bias can creep in. For example, if the models are trained on usage patterns of Western audiences, they may not perform with as much accuracy for more localized experiences in Asia.”
This kind of bias can have serious implications, especially for global companies that serve diverse populations. To mitigate this risk, data teams must ensure their training datasets are diverse and inclusive, covering a range of demographics and regions. They should also conduct regular audits of their models to identify and correct any bias that could skew outcomes.
Learn more in Product School’s guide to AI for enterprises.
Unlocking Business Potential with AI-Powered Data Insights
The world of AI data analytics is rich with opportunities for business leaders to make better, more informed decisions. By leveraging AI tools for data analysis, companies can gain deep insights from their data, identify areas for improvement, and act decisively to enhance customer experiences and operational efficiency. AI can transform a massive amount of raw data into clear, actionable insights, allowing businesses to move quickly and strategically in a competitive marketplace.
However, successful implementation requires more than just adopting the latest technology. As discussed above, the "just add AI" mindset can end up doing more harm than good. Instead, business leaders should focus on selecting the right solution for each specific problem, ensuring ethical considerations like data privacy and algorithmic bias are addressed, and maintaining a balance between AI-driven insights and human oversight.
As AI continues to evolve, embracing its power responsibly and effectively will be the key to driving sustained business growth. Leaders who understand how to best use AI for data analytics will be in the best position to navigate the complexities of their industries, innovate continuously, and build a culture of data-driven excellence.
AI Training for Teams
Accelerate revenue and 2x productivity when you equip teams across your organization with AI skills and training.
Learn MoreA special thanks to Ash Sharma for generously sharing his thoughts on this important topic!
Updated: October 28, 2024