The Product Management Blog
By sharing your email, you agree to our Privacy Policy and Terms of Service
Featured
Discover our posts
Showing 9 of 572
Leadership
Team Effectiveness: We Cracked the Code so You Don’t Have To
Understand key factors, models, and frameworks to boost team effectiveness. Learn how to improve team performance with proven strategies.
Product Fundamentals
24 Product Management Tools: The Ultimate List for 2025
Find the best product management tools to streamline workflows, improve collaboration, and build better products — featuring expert picks and insights.
Product Strategy
Organizational Agility and Why It’s Crucial for Success
Discover what makes an organization agile, the impact of organizational agility on performance, and how to measure and improve it.
Product Strategy
The Product Development Process: A Step-by-Step Guide
Learn the product development process step by step — from idea to launch. Discover key stages, best practices, and tips for faster iteration.
Product Strategy
9 Types of Prototypes You Should Know About
Learn about types of prototypes — low-fidelity, high-fidelity, functional, interactive, and more — and how they help refine product ideas before launch.
Career Development
Product Strategist: Role, Skills, & Career Path
Discover what a product strategist does, key skills, salary, and how the role compares to product managers, marketing strategists, and design strategists.
Product Strategy
What Is a Product Goal? Definition, Examples & Best Practices
Learn what product goals are, how to set them, and why they matter. Get expert tips, examples, and frameworks to drive real product success.
News
Product School Membership: The All-Access Pass to Career Growth
Supercharge your product career with Product School membership: unlimited access to expert-led certifications, plus 1:1 career coaching.
Digital Transformation
Agile Digital Transformation: What It Is & Why It Works
Find out how Agile digital transformation accelerates innovation, reduces risk, and drives business success with flexible, iterative strategies.
Subscribe to our newsletter
A newsletter that will make you a better Product Manager
By sharing your email, you agree to our Privacy Policy and Terms of Service
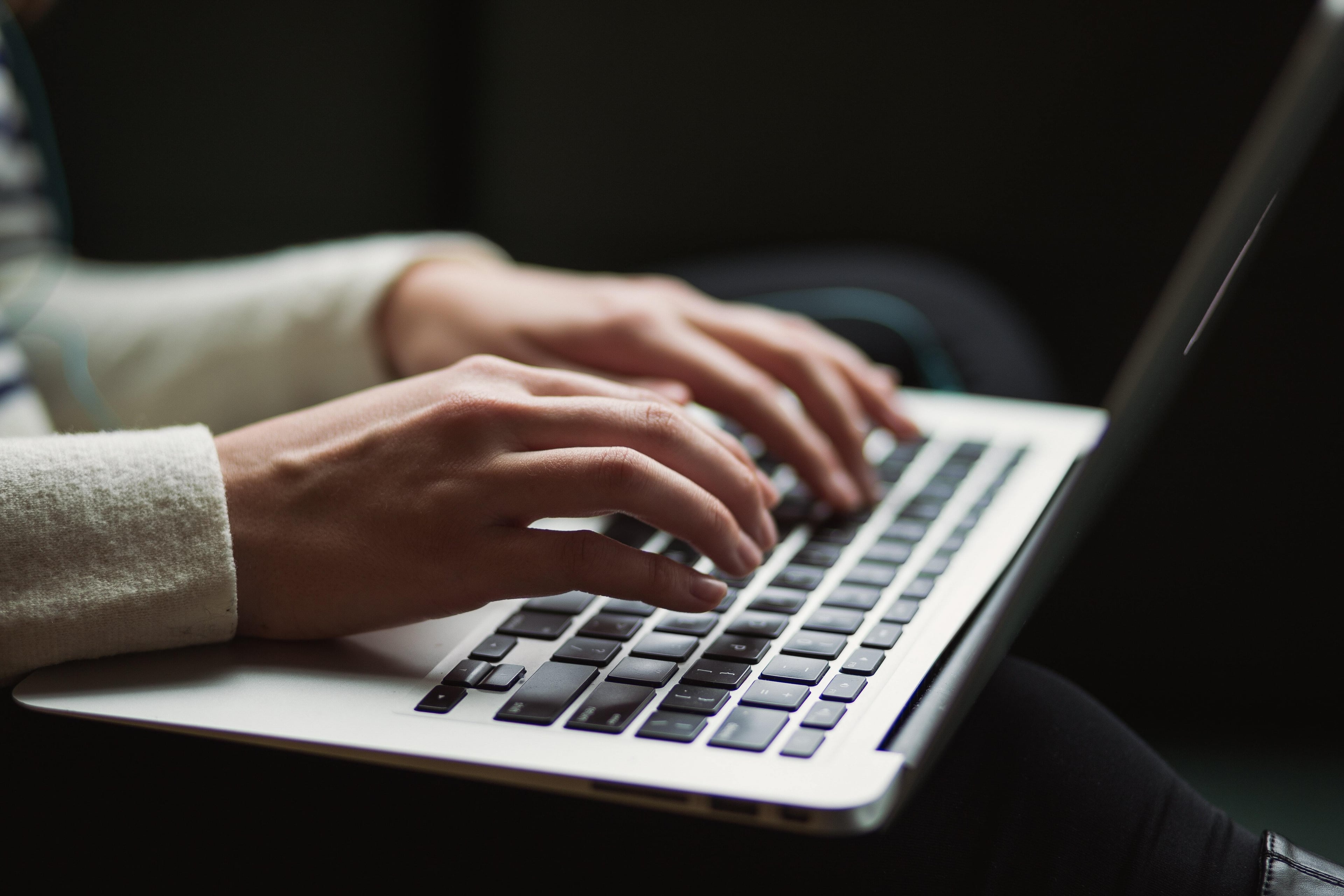